Overview
Although we tend to think of evolution as something that happens slowly and over long periods of time, it is actually happening around us all the time. In this session, Drs. Turner and Malik focus on the ability of viruses to rapidly evolve and adapt to changing environments. Turner explains how some viruses have evolved to infect many hosts while others infect a single cell type. He also uses experimental evolution to study the ability of a virus to survive under different environmental conditions versus its ability to effectively reproduce. Malik studies the ongoing battle between viruses and their hosts and describes some of the tricks each use to survive attacks by the other.
Introduction to Virus Ecology and Evolution
Concepts: Biology of viruses, reproducton and adaptability of viruses to environmental challenges
Note: This video is optional for this session.
00:00:14.17 Hi.
00:00:15.17 I'm Paul Turner.
00:00:16.17 And today I'd like to talk about the fundamentals of virus ecology and evolution.
00:00:20.10 This is not designed to be a comprehensive overview, but rather an introduction to the
00:00:24.20 very many ways that viruses interact with other organisms, and how they evolve on the
00:00:29.01 planet.
00:00:30.03 I'm currently the Chair and full professor of Ecology and Evolutionary Biology at Yale
00:00:35.20 University, and I also have an appointment in the Microbiology program at Yale School
00:00:40.20 of Medicine.
00:00:42.13 So, we can begin with a very fundamental question of, exactly what is a virus?
00:00:48.15 There are many ways of depicting the relationships between organisms on the planet as a way to
00:00:54.16 catalog biodiversity and evolutionary trees are generally the way that we use, within
00:01:00.07 evolutionary biology, a depiction of relatedness among biological species and groups.
00:01:04.21 So, for example, this is a depiction of the Tree of Life, but it's actually a pretty misleading
00:01:11.04 one.
00:01:12.04 Although many of the organisms are recognizable, here, they're really mostly drawn from only
00:01:16.00 one domain of life, the eukaryotes.
00:01:18.03 These are the most recognizable animals, plants, etc that most people are familiar with.
00:01:24.14 Instead, a more accurate depiction might be something like this wraparound tree, where
00:01:29.12 depicted in purple are the very many bacterial species, in this particular tree, that have
00:01:34.10 a name.
00:01:35.22 And another domain, shown in green, are the archaea which are, like the bacteria, single-celled
00:01:42.01 species, and they are more often found in extreme environments.
00:01:46.06 But you'll note in this tree that the archaea are close relatives to the groups with which
00:01:50.13 we belong, the eukaryotes.
00:01:52.23 So, for your reference, here are you on the tree.
00:01:57.00 And this Tree of Life also is a bit misleading in the sense that it cannot catalog the amazing
00:02:03.01 biodiversity of another very important group of biological entities on this planet, and
00:02:08.06 those are the viruses.
00:02:10.05 So, these three domains of life comprise only cellular life, and viruses are different because
00:02:16.07 they are not cellular.
00:02:18.00 At the top of this diagram, we see very familiar body plans of eukaryotes, including something
00:02:22.23 like a blue whale, a sequoia tree, and even single-celled eukaryotes are very common on
00:02:28.06 Earth.
00:02:29.10 The bacteria and the archaea, as stated earlier, these are single-celled organisms, so even
00:02:35.12 though many of them are close relatives -- closer than the bacteria than within the archaea
00:02:39.14 -- the body plans of bacteria and archaea are very simple in that they have only a single-celled
00:02:44.16 body plan.
00:02:45.16 Now, if we take a closer look at what the body plan is of a typical virus, it has a
00:02:50.18 protein shell as well as nucleic acid, either RNA or DNA, that's protected from environmental
00:02:56.05 degradation by this protein shell that has a very special name, called a capsid.
00:03:01.04 So, at the top we see, here, the influenza virus, which is a very typical virus that's
00:03:06.20 recognizable to most people by name, and it's shown that it's relatively a circular virus,
00:03:12.19 with the RNA as the nucleic acid and that's protected from the environment by a capsid,
00:03:18.04 whereas something like a phage, phage T4, this is a short form of the name bacteriophage,
00:03:23.14 which translates to eater-of-bacteria.
00:03:25.18 These are viruses that are specific to prokaryotes, and this phage, you notice, has a much more
00:03:31.23 elaborate body plan than an influenza virus.
00:03:36.05 But what they both have in common is nucleic acid that is protected from the environment
00:03:40.23 by a protein shell called a capsid.
00:03:43.08 A typical virus life cycle looks like this, in its most simple form.
00:03:48.03 As long as a virus attaches to some cell, that it has the proper protein binding recognition,
00:03:54.09 there's a possibility that it will get in, or that at least its nucleic acid will enter
00:03:59.07 the cell.
00:04:00.07 And, at this point, the primary mission of a virus is to hijack the metabolism of that
00:04:05.11 cell and divert those energy sources towards reproduction of viruses rather than normal
00:04:12.00 cellular function.
00:04:13.18 At this point, the particles can get to maturity within the cell and they can be released,
00:04:18.15 and go on and infect other cells.
00:04:21.10 So, this is very much a generalization, but it's a good way to think about the fundamentals
00:04:26.22 of how viruses replicate and reproduce.
00:04:29.19 So, now, you can really think of biological entities, perhaps life if you want to define
00:04:36.09 viruses as living, as being split into two basic body forms: the capsid-encoding organisms...
00:04:44.00 you can think of these as all viruses because they share the properties of nucleic acid
00:04:49.08 surrounded by a capsid, and the viruses themselves would be specific to either the archaea, the
00:04:54.24 bacteria or the eukaryotes; and ribosome-encoding organisms, the cellular ones, are quite different.
00:05:01.18 They are related to each other and they are the three major domains of bacteria, archaea,
00:05:07.09 and eukaryota.
00:05:08.20 So, the major mystery in all this is whether they all trace back to the same route.
00:05:15.08 This is really an open question in virology.
00:05:18.04 It deals with the evolution of virus origins and it's really unclear how viruses first
00:05:23.01 occurred on this planet, when did they first evolve, and, especially, whether they evolved
00:05:27.14 on the planet preceding cellular life.
00:05:30.24 One major idea that is popular, but is by no means the only idea of how viruses first
00:05:35.18 arose on the planet, is that they might have actually predated the evolution of all cellular
00:05:40.11 life.
00:05:41.19 And there's a key bit of data that supports this idea, in that, if you look at virus genes
00:05:48.03 and compare them in their genetic sequence to cellular life, that is available in genetic
00:05:53.10 databases, you often find a mismatch.
00:05:56.01 So, that suggests that viruses are not simply some more fundamentally simple version of
00:06:03.05 cellular life; instead, they might be something really fundamentally different that might
00:06:07.19 have predated cellular life altogether.
00:06:09.22 So, LUCA, here in this diagram, stands for last unique common ancestor, which is the
00:06:15.01 shorthand version of everything that cellular life perhaps traced back to.
00:06:19.22 And this diagram is indicating that there might have been an ancient virosphere, for
00:06:24.00 which all the viruses that are infecting the archaea, the bacteria, and the eukaryotes
00:06:30.14 existed alongside in this time period or even predating LUCA, and that, today, we see this
00:06:35.21 amazing biodiversity of virus types, but they might have been here billions of years ago
00:06:41.11 and even before cellular life.
00:06:44.02 So, the rest of the talk will feature several lines of evidence that suggest that viruses
00:06:51.08 just might be the most successful of Earth's inhabitants.
00:06:55.09 So, I'll go through five lines of evidence that really support what I would assert as
00:07:02.07 the extreme biological success of viruses relative to other entities on the planet.
00:07:07.12 The first will be growth potential; next, we'll talk about abundance, biodiversity;
00:07:12.13 adaptability; and, perhaps most important, it's very evident that viruses have huge impact
00:07:18.20 on other organisms on this planet.
00:07:22.09 First of all, growth potential... because there are so many different ways of cataloging
00:07:26.13 and determining what biological... biological success even means, let's begin with just
00:07:31.10 the sheer ability to grow, to reproduce, and produce copies of oneself.
00:07:37.01 So, growth potential can be pretty amazingly impressive for a lot of organisms.
00:07:44.09 Some eukaryotes, our close relatives, are able to reproduce very quickly, such as the
00:07:49.00 fruit fly has a very short developmental cycle, such that progeny are produced in a very short
00:07:54.13 period of time.
00:07:55.13 But, really, the abundance of the number of organisms that are... that can be produced
00:08:02.06 through reproduction... amphibians, they are also pretty impressive.
00:08:06.12 They can sometimes lay hundreds or thousands of eggs that will successfully develop eventually
00:08:11.12 into adults.
00:08:12.12 Now, the bacteria and the archaea, I would say, are even more impressive.
00:08:16.00 They undergo a form of reproduction called binary fission where each cell separates into
00:08:21.07 two daughter cells.
00:08:22.11 So, in this way they can grow very, very quickly, as long as there are abundant resources available.
00:08:27.11 So, I would say the bacteria and the archaea, on average, grow much faster than most eukaryotes
00:08:33.14 that we would consider.
00:08:36.14 All eukaryotes, bacteria, and archaea have genetic inheritance across generations that's
00:08:42.08 through DNA as the nucleic acid.
00:08:44.18 So, they sometimes do have this amazing growth potential, but let's keep in mind that the
00:08:49.09 only way that traits pass across generations is through double-stranded DNA inheritance.
00:08:56.06 Considering the viruses, they grow much, much faster than most bacteria, archaea, and eukaryotes.
00:09:02.18 So, the ability for viruses to enter a cell, hijack the metabolism, and make progeny can
00:09:09.06 sometimes lead to hundreds or thousands of particles exiting that cell.
00:09:14.08 So, this would be the case for bacteriophages that are infecting the bacteria and the archaea,
00:09:20.11 as well as viruses of eukaryotes.
00:09:22.17 If they infect a tissue, for example, in the human body, there can be an amazing capacity
00:09:27.04 for each one of those infected cells to produce upwards of 10,000 or more virus particles
00:09:33.13 per cell.
00:09:35.02 Also, in relation to inheritance across generations, I would say that the viruses have obviously
00:09:41.16 more options for how this occurs in the natural world, whereas the bacteria, archaea, and
00:09:48.00 eukaryotes are confined to largely DNA inheritance through double-stranded nucleic acid, both
00:09:53.23 double-stranded DNA and double-stranded RNA are possibilities for the nucleic acid inheritance
00:09:59.03 in viruses, as well as single-stranded forms of those nucleic acids.
00:10:03.17 So, essentially, viruses are covering the entire gamut of what is possible on this planet
00:10:08.17 for modes of inheritance through genetic... through genetic means.
00:10:12.15 So, now let's move on to talking about virus abundance.
00:10:16.11 And this is very amazing and, when you look at the numbers, you'll see that viruses easily
00:10:22.04 outnumber all other biological forms on this planet.
00:10:25.23 We'll begin with saying that viruses are very abundant and we know this because if you look
00:10:31.14 in natural habitats on Earth, sometimes these support cellular life and there's actually
00:10:36.23 an amazing capacity for cellular life to thrive in deep-sea vents and complete darkness, where
00:10:43.03 there are other forms of resources than the sun to produce progeny, ultimately, when you
00:10:48.20 look at very dry and hot regions, cold regions, atmosphere, soil... everywhere you find on
00:10:55.24 this planet the possibility of cellular life existing, you see alongside it viruses.
00:11:02.15 And the key thing here is that viruses generally outnumber cellular entities ten to one in
00:11:08.09 each of these environments.
00:11:10.09 Therefore, you can make some rough calculations and estimate, across this world, at any one
00:11:16.12 period of time, an estimate of some 10^31 particles of viruses in any instant is a very
00:11:23.02 reasonable one for the viruses on this planet.
00:11:26.23 And we all realize, hopefully you can remember, that nucleic acid exists in a compacted form
00:11:33.14 within cells and also within capsids of viruses, but if you just take these virus genes and
00:11:39.22 genomes, and you unravel them so that they're at their full length, and you lay them end
00:11:44.12 to end, off of the Earth's surface, this would extend to 250 million light years away, which
00:11:49.19 is an incredible distance.
00:11:51.15 That would allow you to reach the Perseus cluster, which is pretty far from Earth.
00:11:56.18 So, now we've talked about the amazing growth potential and the sheer abundance of viruses
00:12:03.01 on this planet, already these are two impressive ways of indicating that biological success
00:12:08.15 of viruses is just amazingly impressive, but now let's look at something that's largely
00:12:13.09 hidden to view.
00:12:15.21 Biodiversity is something that's fascinated humans for a long period of time, probably
00:12:19.11 ever since humans have observed other organisms around them.
00:12:24.02 But there's an invisible kind of biodiversity on this planet, especially in the virus world,
00:12:29.02 that has only been seen relatively recently with the kind of tools and machinery such
00:12:32.23 as electron microscopes that let us glimpse these larger number of entities that surround
00:12:38.10 us on the planet.
00:12:40.16 The first thing to state is that viruses by no means are identical to one another in their
00:12:45.13 morphologies.
00:12:46.13 Some of them are amazingly complex, with beautiful body plans that almost look like a lunar lander
00:12:51.18 -- something incredibly beautiful, I would say, in nature, but often escapes our attention
00:12:56.23 because they are so small.
00:12:59.01 Other forms of viruses are more like tubes and beautiful icosahedrons, so there's an
00:13:05.12 amazing variety, but you'll notice that the line here at the bottom...
00:13:10.08 0.5 micron, which is 1/2000 of a millimeter... all of these body plans that I'm showing you
00:13:16.07 thus far suggest that viruses are submicroscopic.
00:13:20.14 And I would say that's an amazing amount of beautiful morphology, complexity, and biodiversity
00:13:26.08 that is also amazingly small in size, so I think that that's pretty impressive.
00:13:31.15 But there's a little quirk to viruses and the way that they had been isolated from natural
00:13:36.08 sources through time, that researchers actually missed the fact that some viruses are not
00:13:42.02 submicroscopic.
00:13:43.02 In fact, some of them are actually larger than the smallest-size cells.
00:13:47.19 So, newly discovered viruses, I would say, are macroscopic -- certainly when you consider
00:13:52.24 a virus like mimivirus relative to the size of an E. coli cell.
00:13:58.04 Here's something more typical of what someone would continue... would... would think of
00:14:01.16 as a typical particle size, and that would be HIV.
00:14:04.18 So, the point here is that by no means are all viruses submicroscopic.
00:14:09.16 Instead, some of them rival or exceed the size of cellular life.
00:14:14.08 Now, these mimiviruses did escape our attention through a little quirk of how people isolated
00:14:20.00 viruses from nature, putting them through filtration and assuming that anything that
00:14:24.04 passed through a very small pore size must be a virus, whereas everything that was held
00:14:28.15 back must be cellular or cellular debris.
00:14:31.23 But all one has to do is increase the pore size in these filtration experiments and you'll
00:14:37.08 find that some amazingly large-sized viruses are evident and still thriving on this planet.
00:14:43.14 So, you can go off the coast of Chile, for example, and within sea water you could find
00:14:48.04 these large-sized viruses.
00:14:50.07 It's also available to see them in ice cores in places like Siberia, where researchers
00:14:56.23 very carefully can take old material and bring it up to the Earth's surface, some 30,000-year-old
00:15:03.10 ice cores have been brought up to the Earth's surface.
00:15:05.12 And, within these, we see these large-sized viruses.
00:15:08.22 That alone indicates that large-sized viruses are certainly not anything new; they've been
00:15:13.18 around on this planet for some period of time and they merely escaped our attention through
00:15:17.21 quirks of methodology.
00:15:21.02 And the natural history study of viruses is something that I would say is certainly thriving.
00:15:26.19 There are entire research programs that can go into places like Yellowstone Park in the
00:15:31.09 USA, where there are certain extreme environments, especially hot springs, and one can look for
00:15:37.07 viruses that are infecting some of these extremophiles.
00:15:41.14 And it's very easy to find that these viruses are completely new to virus family classification.
00:15:49.08 We see body forms and, ultimately, when one characterizes these viruses through genetics,
00:15:54.12 they are not at all closely related to other virus families that have been described in
00:15:58.16 the past.
00:15:59.16 So, I would say that natural history is alive and well in virology, and it is possible to
00:16:04.16 go out and very easily, in nature, isolate material that shows you very new viruses that
00:16:10.09 are entirely new to the biological classification.
00:16:14.00 Adaptability... so, this is the ability for organisms to interact with their environment,
00:16:21.04 to undergo selection to become better adapted through time, so that they create a better
00:16:26.00 match to their environment.
00:16:28.06 Darwin was the one who best articulated this through the process of natural selection,
00:16:32.15 so we know... we will now talk about how viruses have an amazing capacity, on average, to adapt,
00:16:38.05 and this certainly contributes to their biological success on the planet.
00:16:41.18 So, Darwin had a way of explaining natural selection that was beautifully elegant.
00:16:47.07 Essentially, if you have these four components, you could think of natural selection occurring
00:16:51.15 in any biological system with inheritance, no matter whether it's on Earth or when we
00:16:56.12 ultimately, probably, will discover life elsewhere.
00:16:59.01 So, if the individuals in a population vary from one another, and if that variation is
00:17:04.12 heritable -- if it could be moved across generations -- one can expect that some of those variants
00:17:09.21 are going to be better advantaged than others in surviving or reproducing, according to
00:17:14.01 whatever the details are of the environment or the ecology that they... that they experience.
00:17:19.05 So, ultimately the population should change genetically, or evolve, to reflect the ones
00:17:26.21 that are the most successful variants.
00:17:29.03 And if all of this is in place, then evolution by natural selection can occur.
00:17:33.24 Darwin best articulated this idea in his most famous book, On the Origin of Species.
00:17:39.23 A great example of this comes from the virus world, where HIV is of course a very important
00:17:46.02 biomedical problem that humans face, HIV that can ultimately progress to AIDS through infection.
00:17:53.00 But not too long after the AIDS epidemic occurred there was a wonder drug called AZT that people
00:17:59.22 thought would be the thing that would just eliminate this problem.
00:18:04.19 Without going into the details, the ability of AZT is to disrupt HIV's capacity as an
00:18:10.23 RNA virus to enter a cell, replicate to turn into a DNA form that inserts into the genetic
00:18:17.18 material, and then exits the cell again as RNA.
00:18:21.00 And this lifestyle in viruses we call a retrovirus.
00:18:25.24 So, here's the problem with treating HIV as an RNA virus that has an amazing mutational
00:18:33.00 capacity with only a single drug.
00:18:35.18 Ultimately, we would expect that drug to fail and unfortunately we only observed this when
00:18:40.15 AZT became popular and used as a drug, and it was seen to widely fail through time.
00:18:46.00 So, through mutation, virus populations can change, especially RNA viruses, which have
00:18:52.13 no ability to correct the errors that just simply occur in the replication process.
00:18:58.16 In this diagram, you see how an infection could begin with a HIV population that is
00:19:04.06 identical to one another, and would be entirely susceptible to AZT.
00:19:09.20 And through time, because of this error ability in the virus population, you are going to
00:19:15.18 have partially resistant or fully resistant forms of the virus simply show up, and these
00:19:21.23 would be resistant to AZT, either partially or completely, as shown in the diagram.
00:19:27.05 And importantly, keep note of this: all of that variation occurs before any AZT is brought
00:19:35.12 into the human patient.
00:19:37.02 So, ultimately, what happens is very similar to what we see with evolution by natural selection
00:19:43.12 -- in fact, it's identical and it's a great description of it.
00:19:46.02 The individuals vary, the variation is heritable, some of those variants are advantaged when
00:19:52.06 AZT eventually is the ecological challenge that the population experiences, and then
00:19:58.07 ultimately those variants take over the population, or it evolves, so that the entire population
00:20:04.23 has escaped AZT as the drug that would ordinarily, we would hope, control it.
00:20:09.21 So, unfortunately, this was observed in every single patient who was administered AZT.
00:20:14.13 It either happened very early on if those mutations occurred early, or later on, if
00:20:19.11 they just happened to occur later.
00:20:21.06 But ultimately, the unfortunate outcome is that AZT always failed.
00:20:27.02 HIV and other disease scourges of humans that are caused by virus... virus infections are
00:20:34.17 not new.
00:20:36.02 Many of them we can find evidence of having occurred in human populations long ago.
00:20:40.18 When one looks at hieroglyphics, mummified remains, artwork from older populations of
00:20:47.03 human from ancient times, this reveals the antiquity of virus diseases.
00:20:52.01 For example, this man, shown in a heiro... in a hieroglyphic from ancient Egypt around
00:20:57.00 3700 BC, has a very characteristic clubfoot that's associated with poliovirus infection
00:21:05.08 that entered in the gut, like all polio viruses ultimately do, but then sometimes poliovirus
00:21:10.18 moves to your neural system, and it can disrupt your normal development and lead to limb deformities.
00:21:16.13 So, this man is definitely showing a characteristic deformed limb of a poliovirus infection.
00:21:24.21 Smallpox is another old disease of the human population, and this artwork is showing an
00:21:30.18 unfortunate person suffering from a smallpox infection, which would be ultimately lethal.
00:21:36.15 And, fortunately, we don't worry about smallpox anymore because there was a global vaccine
00:21:41.04 campaign that eliminated it as a natural thing that would infect humans, but the point is
00:21:46.14 that we can find, through this ancient material that humans and older populations have left
00:21:51.09 behind, that humans have been suffering virus diseases for a very long time.
00:21:56.03 I would assert that, probably, this has happened as soon as humans evolved as a species on
00:22:01.11 the planet, there would be viruses that would challenge their health.
00:22:05.07 So, I'd like to end with talking about the impact, in general, that viruses have on other
00:22:10.13 organisms on this planet, and this is really quite profound.
00:22:15.21 Similar to the examples I just covered for smallpox and HIV, etc, there are many diseases
00:22:23.03 that humans face that are a challenge to our health and our mortality, and a lot of these
00:22:28.08 are endemic problems, for example, the parasite that is transmitted by a mosquito and causes
00:22:34.20 malaria in sub-Saharan Africa and other tropical regions.
00:22:38.07 This has been around for a very long time -- that happens to be a eukaryote -- and you
00:22:41.24 could see the response, even in our genetics, of ways that we would be naturally better
00:22:47.06 able of fighting off malaria, and it has contributed to ways that the human population has changed
00:22:52.10 genetically through time.
00:22:54.03 But that's different than an epidemic that is highly deadly and rises in frequency very
00:23:00.24 quickly, and impacts a lot of, in this case humans, through mortality, something that
00:23:06.08 happens very quickly.
00:23:07.13 So, here are four examples, and I'm highlighting, in red, that three of them were due to viruses,
00:23:13.23 virus illnesses.
00:23:14.23 So, the Spanish influenza of 1918, amazingly, before humans were traveling around the world
00:23:21.11 through flight, through commercial flight, still 50 to 100 million people around the
00:23:26.13 world contracted influenza and died from this highly virulent form of the virus.
00:23:33.24 More recently, the HIV/AIDS epidemic that started around 1981 is something that has
00:23:38.15 unfortunately left roughly 35 million people dead and many, many more than that are infected.
00:23:44.01 Fortunately, we have new ways, things other than AZT, that keep people alive for a long
00:23:48.22 period of time when they're HIV-infected, but certainly during the early part of that
00:23:53.05 pandemic we saw a high degree of human mortality and we still see it today.
00:23:57.20 Now, it's hard to put exact numbers on the New World smallpox epidemic, around 1520,
00:24:03.11 when this started.
00:24:04.18 This is when Europeans came to the New World, interacted with Native American populations,
00:24:09.21 in some places displacing them, and they introduced into those populations smallpox, something
00:24:16.08 that Native Americans had not seen.
00:24:18.09 So, this was experienced as a highly virulent illness in Native American populations.
00:24:24.02 It's unclear how many people died because we just don't have accurate records of how
00:24:27.12 many people were in these populations at the time.
00:24:30.00 Certainly, this could have been on the order of the two pandemics that I listed above.
00:24:36.07 And last, I will remind you that some bacterial illnesses also have the capacity to have huge
00:24:42.22 impact on human populations.
00:24:44.20 The "Great Plague" in Europe in the middle of the 1300s was something that left in its
00:24:49.06 wake some 28 million people, or roughly 40% of the European population succumbed during
00:24:55.09 the Great Plague.
00:24:56.21 It's a little controversial how this bacterial illness could account for that amount of mortality.
00:25:03.09 Some people believe that the sheer poor living conditions that people experienced at that
00:25:07.10 time left them highly vulnerable to something like virus illnesses, as well, that might
00:25:12.16 have contributed to those millions of people who died.
00:25:15.00 So, again, we're a little uncertain there, but for the most part these examples are grim
00:25:20.05 ones of how impactful viruses can be in terms of mortality.
00:25:24.10 So, I don't want to leave you with all bad news about viruses, because, certainly, there
00:25:29.15 are a lot of viruses that positively impact other organisms, including humans.
00:25:35.18 So, let's begin long, long ago, some billions of years ago when the cyanobacteria appeared
00:25:41.14 on this planet and they started to thrive through evolving photosynthesis, that, 3 billion
00:25:47.21 years ago or more, this changed the Earth's atmosphere so that 50%, today even, of the
00:25:54.16 Earth's oxygen is due to photosynthesis that comes from cyanobacteria living in the ocean.
00:25:59.23 And if they hadn't evolved long ago and changed the average amount of oxygen in the atmosphere,
00:26:05.18 it would have been impossible for large-bodied organisms like us to even evolve in their
00:26:09.21 wake.
00:26:10.21 So, we can thank the cyanobacteria for that.
00:26:13.22 But there's an interesting thing that most people don't know and that is cyanobacteria
00:26:18.23 undergo a lot of mortality from cyanophages.
00:26:22.12 These are viruses that live alongside them in the ocean and they outnumber the cyanobacteria
00:26:28.18 and they thrive on the cyanobacteria, essentially as resources.
00:26:32.24 But there was an interesting finding not long ago that these cyanophages have genes that
00:26:38.18 allow for photosynthesis to occur.
00:26:40.24 And that was a very strange discovery, because we know that viruses don't undergo metabolism.
00:26:46.18 What would they be doing with photosynthesis genes?
00:26:49.10 It starts to make more sense when we think about the virus life cycle and the necessity
00:26:53.19 of keeping the cell going and churning through its metabolism, so that viruses can undergo
00:26:59.15 proper replication.
00:27:00.23 Therefore, it's not surprising that they bring these genes into the cell with them, so that
00:27:06.01 those processes can occur.
00:27:08.08 But a factoid I'll leave you with is that perhaps 5% of the breaths that you'll take
00:27:13.03 today ultimately come from those genes that are on cyanophages, rather than any other
00:27:18.12 source of oxygen.
00:27:21.20 We are more and more familiar with microbiomes -- you hear more and more about this in the
00:27:26.04 news.
00:27:27.04 So, these are the fungi, the bacteria, and the viruses that live on and in macroorganisms
00:27:31.23 such as humans.
00:27:33.14 And it's really interesting how these communities vary, even through locations in our body.
00:27:38.24 Inside and outside, you have different communities of these microbes thriving on and in your
00:27:43.08 body.
00:27:44.08 Well, the same thing goes, but different composition of those communities exists, for animals,
00:27:50.01 plants, and other macroorganisms on the planet.
00:27:53.13 Some of these we keep in our homes or we rely on for food, so it's important to think about
00:27:58.11 how these interactions are occurring with organisms that are essential to us, or that
00:28:04.15 simply give us pleasure, like as pets in our home, they have their own characteristic microbiomes
00:28:09.07 that we're learning more and more about.
00:28:11.19 And I should also emphasize that, certainly, all wild animals and also wild animals that
00:28:17.13 we rely on for food, they have their own characteristic microbiomes too.
00:28:22.13 So, viruses in your microbiome... you hear in the news about how the microbiome is probably
00:28:30.12 the thing that is causing certain traits in humans, making... making us more disease-prone
00:28:35.24 or disease-averse, so you'll hear a lot more about this in the coming decades, but you
00:28:41.13 actually don't hear that much about your virome, the component of your microbiome that is the
00:28:46.11 viruses.
00:28:47.14 There was a neat discovery recently that, perhaps, these viruses that exist on and in
00:28:53.07 your body are interacting with your body to make you healthier.
00:28:56.19 This diagram is showing how phages, which cannot infect our cells, can interact with
00:29:03.06 the mucus layers that we naturally produce that help protect us as a barrier from bacterial
00:29:08.16 infection.
00:29:09.22 Interestingly, these phages will interact with the mucus layer and be oriented in a
00:29:15.11 way such that their tail fibers are able to interact with any bacteria getting to the
00:29:20.05 mucus layer and trying to transit through to get to your cells.
00:29:23.21 This provides an extra barrier that protects you against those bacteria, because the phage
00:29:29.03 will attach, replicate, kill the bacteria, exit the cell, and more of those infection
00:29:35.10 events can occur.
00:29:36.19 This is certainly to our benefit, but we have a lot more to learn about whether this is
00:29:40.20 something that has been an... an adaptation that evolved for these phages to interact
00:29:46.03 with macroorganisms to do this, or whether they're simply taking advantage of the fact
00:29:50.11 that phages are everywhere, sometimes they are in the correct place for this to happen.
00:29:57.19 So, a final thing that I'll leave you with is that the human genome project gave many
00:30:04.13 surprising results, but there was a very surprising one in terms of, if you look at our DNA com...
00:30:09.24 our... our... our DNA chromosomes, you'll find a nice match between roughly 8% of your
00:30:16.15 human DNA seems to be viral-derived.
00:30:19.19 And one might ask, well, how is that possible?
00:30:22.17 You can think of this as the ghost of infections past.
00:30:25.08 In our long ago species ancestors that led to humans, ultimately, there were some virus
00:30:31.17 infections that those organisms suffered and interestingly there can be viruses that avoid
00:30:39.10 detection by their hosts because they have special genes that make them hide, so, essentially,
00:30:45.17 what has occurred in the evolution of placental mammals is that we could not have existed
00:30:50.06 on this planet unless, long ago, our ancient ancestors had undergone these virus infections,
00:30:57.21 and those genes, instead of becoming deleterious and helping viruses avoid detection by our
00:31:03.22 long ago ancestors, instead they were genes that were co-opted and taken into our genome,
00:31:09.15 and ultimately helped placental mammal mothers not reject the baby in the body.
00:31:16.05 And this is an amazing thing due to what are called syncytian proteins and genes that come
00:31:20.01 from viruses.
00:31:21.14 This is clearly a way that virus genes can be highly useful to other organisms and, in
00:31:27.18 fact, there's no way that you would be sitting here listening to me give this talk unless
00:31:32.02 these ancient infection events had occurred, and had led, ultimately, to the evolution
00:31:36.15 of placental mammals.
00:31:37.24 So, I've covered a lot of evidence for what we would say indicates that viruses are the
00:31:45.16 most biologically successful entities on this planet.
00:31:49.12 If you look at their growth potential, their abundance, and their biodiversity, they simply
00:31:54.15 outnumber, and can grow faster, and produce a larger number of forms than any other organisms
00:32:02.02 that we know of.
00:32:03.19 In addition to that, they can interact with other organisms in their environment, and
00:32:08.01 have an amazing capacity to adapt to environmental change, which I find completely fascinating
00:32:13.12 because, essentially, viruses cannot control where they go in the environment, and yet
00:32:18.13 they can encounter environments that are challenging to them and improve through time through adaptation
00:32:24.12 by natural selection.
00:32:26.00 Ultimately, their impact on the rest of the biological world is profound and immense,
00:32:32.12 and literally we thank viruses for some genes that they have devoted and given to us in
00:32:38.03 our genome, and this is something that's clearly impacted -- positively -- the human species
00:32:44.16 through time.
Virus Adaptation to Environmental Change
Concepts: Viruses adaptability to environmental changes
Note: Please watch original video from time 4:45 to 43:36.
00:00:14.24 Hi.
00:00:15.24 I'm Paul Turner from the Department of Ecology and Evolutionary Biology at Yale University,
00:00:19.20 and the Microbiology faculty at Yale School of Medicine.
00:00:23.06 Today, I'd like to present on virus adaptation (or not) to environmental change.
00:00:29.08 This talk describes how viruses have an amazing capacity to adapt to environmental challenges
00:00:35.03 and, yet, we'll find that these champions of adaptation sometimes encounter environments
00:00:40.17 that demonstrate that environmental change can constrain evolution and adaptation, and
00:00:46.13 even these so called champions can face constraint.
00:00:50.11 So, very many challenges exist to viruses in the natural world and you could think of
00:00:56.09 this at all different levels of biological organization.
00:00:59.24 So, if you start at the base level of molecules and cells, the primary challenge for viruses
00:01:06.02 is that they cannot control where they exist in the environment, so they might encounter
00:01:11.04 some cell type and successfully enter if they have the right protein binding to recognize
00:01:16.19 a protein on the cell surface, or they might bump into the wrong type of cell and that
00:01:22.15 protein recognition doesn't occur.
00:01:24.14 So, therefore, it's an immediate and proximate challenge to a virus to infect a cell, depending
00:01:30.02 on where it is in the environment and whether the proper cells exist to infect.
00:01:34.13 In macroorganisms like us, we have tissues composed of different cell types, so if a
00:01:41.00 virus is in your body and it's replicating in one tissue type, it might be challenged
00:01:46.01 to infect a different tissue that's nearby and it's incapable of doing so.
00:01:51.05 Hosts, such as humans, have elaborate and beautiful immune systems.
00:01:56.21 Some of them are adaptive, meaning that they change through time and this is a way of our
00:02:01.08 immune system, in a way, keeping pace with microbial invaders and changing at the same
00:02:07.03 pace that they might evolve through evolution.
00:02:10.00 But viruses and other microbes... when they encounter these immune systems, this poses
00:02:14.23 a challenge for them to continue to infect that host, or that host's progeny, or other
00:02:19.19 susceptible hosts in their env... in their environment, depending on whether those immune
00:02:24.00 systems provide an immediate and successful barrier to virus replication.
00:02:30.10 It's amazing thing that some viruses infect humans and also successfully infect very different
00:02:37.12 organisms that are not at all closely related to us.
00:02:41.11 A great example of this are the arthropods, where many pathogens are vector-transmitted
00:02:47.11 by arthropods such as mosquitoes, including viruses.
00:02:51.14 And this is pretty fascinating, because a virus has to grow within an invertebrate and,
00:02:58.05 for example, in a mosquito, it has to grow in the midgut and eventually get to the salivary
00:03:01.21 glands in order to be present in a bite that puts the virus in the bloodstream of another
00:03:07.04 host to be picked up by another mosquito.
00:03:09.21 That's got to be an incredible challenge for a virus to grow both successfully in an invertebrate,
00:03:14.23 like an arthropod, as well as a vertebrate like you, a human.
00:03:18.18 And, last, we have to remember that global-level ecosystem changes affect all biological entities
00:03:25.04 on this planet, including the very smallest ones such as viruses.
00:03:29.02 So, when you think about challenges like climate change and global warming, you have to remember
00:03:35.11 that this is something that is felt by all biological entities, and therefore viruses
00:03:40.06 can also feel the challenge of an ever-warming world.
00:03:43.23 There are many different virus study systems that my group examines.
00:03:48.11 So, these examples are shown in the very many beautiful forms behind me.
00:03:54.09 On the far left, we have vesicular stomatitis virus, which is an example of a single-stranded
00:03:59.15 RNA virus with a negative-sense genome, and in the middle we have a variety of other viruses,
00:04:04.23 also, that will infect eukaryotes, but they happen to have positive single-stranded RNA
00:04:10.05 genomes.
00:04:11.05 Closer to where I'm standing, we have single-stranded DNA filamentous phage, and also double-stranded
00:04:16.17 RNA and double-stranded DNA viruses, in this case, both phages: phage phi-6 and phage T2.
00:04:23.09 So, these are examples within my laboratory of the wide variety of viruses that exist
00:04:28.14 in the natural world, and how a single laboratory can choose to examine this great variety of
00:04:34.11 virus types.
00:04:35.16 Depending on the challenge and the question, we would like to focus on a different study
00:04:39.17 system to examine whether viruses can successfully, or not, adapt to their environments.
00:04:45.21 A big tool that we use that's very popular with others, and a very powerful tool, would
00:04:50.22 be experimental evolution.
00:04:52.24 A way to summarize this method is it's the ability to study evolution in action.
00:04:58.01 So, if you have the right study system in a controlled place, like a laboratory, you
00:05:03.17 can take that population, put it in an environment that you control explicitly, and then examine,
00:05:09.09 how does that population deal with that challenge, both in terms of the traits that it evolves
00:05:14.07 as well as the genotypic changes that it undergoes?
00:05:16.24 So, both phenotype and genotype can be the focus of these studies.
00:05:21.09 An important thing to remember is, even if a researcher is manipulating the environment
00:05:25.20 in the laboratory, it still can be a challenge to a population, and that population can evolve
00:05:32.07 through natural selection.
00:05:33.12 So, you're talking about an artificial environment and yet natural selection can occur.
00:05:38.22 That's because the researcher is not determining which variants in that population will better
00:05:43.17 match the environment; instead, that's due entirely to the mutations and the genetics
00:05:48.15 of that system to meet that challenge or not.
00:05:52.18 And that can happen through the process of evolution by natural selection.
00:05:57.11 A typical design is shown here, where we would begin with some ancestral type.
00:06:03.00 We might be interested, in the case of this hypothetical diagram, in three different treatments
00:06:07.12 that differ in some way in their environmental challenge, and we can track, over the course
00:06:13.16 of generations, how do these independent lineages evolve to meet these challenges?
00:06:20.05 And the nice thing is to include replication in these experiments, such as you can have
00:06:24.22 lineages that are experiencing the same environment, and you can look at how consistently do lineages
00:06:30.23 undergo random mutation, and yet the same mutations might be the ones that rise to fixation,
00:06:36.12 and lead to adaptation.
00:06:38.05 In other cases, there might be different solutions to the environmental challenge, and you'll
00:06:42.20 see divergence between your lineages in the sense that different mutations are meeting
00:06:47.20 the same challenge.
00:06:50.10 Another way to think about these experimental evolution studies is to create a hypothetical
00:06:55.09 diagram of some phenotypic trait that you would want measure -- this might be growth
00:07:00.06 or some other capacity of the system to meet the challenge.
00:07:03.03 So, in this example, I'm illustrating how this trait has some variation at the beginning,
00:07:09.21 and then we could create some sort of an ecological circumstance, or an environmental challenge,
00:07:14.22 in these studies, and, through time, we can keep track of how phenotypes change.
00:07:19.18 So, you'll notice that the average phenotype, along the x axis in this hypothetical example,
00:07:25.18 is shifting to the right, meaning that the mean of the distribution is changing according
00:07:29.23 to which variants are in that population and the ones that are best meeting that challenge.
00:07:34.15 Now, we can go further than a lot of systems, because it's very easy for us in virus studies
00:07:40.04 to take the entire genome from these evolving populations and explicitly look everywhere
00:07:46.08 in the genome for where a mutation might occur.
00:07:48.24 In this case, we can track through generational time, how is the genetics changing in relation
00:07:54.11 to the ecological challenge as well?
00:07:56.15 And this allows a lot of immediate power in making something called a phenotype-genotype
00:08:01.14 association -- you can infer how the changing phenotype is being controlled by underlying
00:08:10.10 genetics, and make some base inferences about what the relationship is.
00:08:15.15 And I don't want to trivialize that because one has to do a lot more work to convince
00:08:19.14 oneself that, perhaps, one mutation is responsible, maybe two or three, or even more complex things
00:08:26.00 can occur like these mutations acting with one another through properties like epistasis.
00:08:31.06 So, this provides an amazing amount of power to examine how evolution occurs according
00:08:37.13 to the environment that you create in these types of studies.
00:08:41.12 The outline for what I want to talk about today is pretty much centered on these two
00:08:45.23 questions.
00:08:47.14 We can consider environmental changes as fostering versus constraining virus adaptation, depending
00:08:54.12 on how the environment is constructed, and these types of experiments are in the natural
00:08:58.18 world.
00:08:59.18 And, especially, now, that takes us to this next question of, are there particular traits
00:09:04.15 that can evolve in viruses that match something intriguing that you see in cellular systems?
00:09:11.06 The investment in survival versus reproduction is often something that's seen as at odds
00:09:16.01 to one another in cellular systems, that you can either invest a lot in survival as an
00:09:21.07 evolutionary trait, but this minimizes your reproductive capacity, or vice versa.
00:09:27.06 So, an intriguing set of studies show that this same constraint, or the same trade-off,
00:09:34.08 can happen even in non-metabolizing organisms, such as the viruses, especially in the viruses.
00:09:42.22 How does environmental change foster versus constrain virus adaptation?
00:09:47.12 Let's look at this question first.
00:09:50.21 Virus emergence is an amazing bio... biomedical challenge that we face today.
00:09:56.01 So, even though RNA viruses, especially, are not that prevalent among the highly prevalent
00:10:01.18 viruses that exist on this planet, they seem to be especially able to jump into new host
00:10:07.20 species and cause harm through disease.
00:10:10.10 So, humans see this through recently emerging pathogens, such as Zika virus, which is sweeping
00:10:17.04 around the globe, and is problematic and creating a challenge to biomedicine, to protect people
00:10:22.16 against Zika virus infection that can disrupt normal development at an early age.
00:10:27.22 A very different example, but still called emergence, is when a virus comes from another
00:10:33.06 species, enters into the human population, and gets locked in and becomes very specific
00:10:39.16 to humans.
00:10:40.16 So, I began with the case of Zika virus, which is not specific to humans, but a great example
00:10:45.17 of a specificity evolution would be HIV, which came into the human population several times,
00:10:51.18 independently, from our primate relatives, especially chimpanzees and certain species
00:10:56.09 of monkeys, and this has led to the evolution of HIV-1 and HIV-2, independently, several
00:11:03.05 times.
00:11:04.06 A third example of emergence would be something that exists both within humans, as well as
00:11:09.21 in other species, and a great example of that would be influenza virus.
00:11:14.09 So, ordinarily, in any year, you can have plenty of the human population seeing flu
00:11:19.03 virus infection and suffering influenza, but what we fear is that there are certain forms,
00:11:24.12 or genotypes, of influenza virus that will be especially virulent and cause a high degree
00:11:29.05 of mortality, and sweep around the globe to infect a lot of humans, and adversely affect
00:11:34.06 human populations, more than a standard flu season.
00:11:37.03 And, especially, we fear that the large reservoir of influenza viruses, that mostly exist in
00:11:43.05 this planet in waterfowl, might lead to a variant that can jump immediately into humans
00:11:48.16 and then be passed from human to human.
00:11:50.23 This would be an example of a flu virus coming from a bird, coming into a very different
00:11:56.00 host species, a mammal, and causing a lot of destruction and mortality because of the
00:12:00.18 inability of the human immune system to deal with the challenge.
00:12:03.20 So, these are three different examples of the same catalogued thing; that thing is what's
00:12:09.20 called emergence, and this is a huge biomedical challenge.
00:12:14.03 We can think of how emergence can or cannot occur for a virus, and that's what I want
00:12:18.09 to focus on next.
00:12:19.11 And there are some certain fundamental expectations, if you have any lineage, whether it's a virus
00:12:25.03 or not, and whether it's encountering an environment that is constant through time, versus changing
00:12:30.21 seasonally, or in a temporal way through time.
00:12:34.22 So, I'm giving two hypothetical examples of this.
00:12:38.11 At the top, we have a hypothetical evolving lineage that sees niche A and niche B in a
00:12:44.22 flip-flopping fashion, and each one of these little circles indicates a generation.
00:12:49.23 So, necessarily, this lineage has to grow in environment A in order to make it long
00:12:55.04 enough in its environment to reach a new environment, B, and so on.
00:12:59.18 Necessarily, we would expect that this... this will select for generalization -- the
00:13:03.18 ability to thrive in both of these environments -- because there is no other option.
00:13:08.06 Now, that's very different than if that lineage has the luxury of seeing only a single environment.
00:13:13.11 In this case, environment A is the only thing it encounters, but I'm underlining the word
00:13:19.18 *tends* to select for specialization, because that's only one possibility.
00:13:24.07 This luxury affords this possibility of being highly specific to your environment and being
00:13:28.23 very good in that environment, but it also is an opportunity for generalization to occur,
00:13:35.15 if you have a correlated response to growing well in other environments.
00:13:39.04 And, essentially, that must be happening in emerging virus pathogens.
00:13:43.16 They happen to have the right genetic capacity that when they jump into a new host species
00:13:48.04 like human, they can just really hit the ground running and grow very well, cause a lot of
00:13:53.15 damage, and ultimately they might be specific to that environment... ultimately, but initially
00:13:59.17 they're highly generalized.
00:14:02.12 We've covered this topic in a variety of papers that I'm listing here that I won't have time
00:14:06.05 to go into much detail, but one can think of this challenge of virus specialism versus
00:14:11.22 generalism happening a lot in the natural world, and it's very easy and powerful to
00:14:17.05 study this in the laboratory, through the experimental evolution method that I mentioned
00:14:21.15 earlier.
00:14:23.14 One system that we've focused on a lot to study how virus specialization versus generalization,
00:14:29.01 and just simply adaptation can happen, is a model known as vesicular stomatitis virus.
00:14:34.17 So, this is a single-stranded RNA virus with a negative-sense genome that's pretty much
00:14:39.21 a workhorse in molecular virology.
00:14:42.06 It's been used for very many decades to understand fundamentals of how RNA viruses infect and
00:14:47.17 replicate in a cell.
00:14:49.05 So, some pictures, here, that I'm showing are just to reflect that we have a lot of
00:14:53.16 prior knowledge for the molecular details of this system, and that's great when you
00:14:58.14 enter into experimental evolution studies, because you don't have to go about measuring
00:15:03.06 that stuff all over again; you can think of the outcome of your experiments in the context
00:15:07.17 of the prior knowledge.
00:15:08.19 So, VSV has a very small genome in size.
00:15:11.21 It has only 11 kilobases in length.
00:15:15.08 And this comprises only 5 genes.
00:15:17.04 So, one can think of this as a pretty simple system.
00:15:20.04 And yet it has a pretty amazing capacity to do things like both reproduce in an arthropod
00:15:26.15 -- it's an arthropod-borne virus or an arbovirus -- and it also can replicate in a mammal.
00:15:31.13 So, in the case of VSV, it's a safe system to use in the laboratory because it might
00:15:36.08 get in a human by accident, but it really doesn't cause much harm.
00:15:40.07 It's agriculturally important in large mammals, domesticated horses, etc, so we do care about
00:15:45.14 it from a disease standpoint, but it's a great, powerful system to use in the laboratory,
00:15:51.04 safely.
00:15:52.04 It comes from the family rhabdoviridae, which also features rabies virus.
00:15:58.04 Here's a summary of some of the data from one of our experiments, where we harnessed
00:16:03.06 experimental evolution to examine, how does this virus deal with a constant environment
00:16:09.11 versus one that is changing through time in that temporal heterogeneous way that I outlined?
00:16:14.10 So, this is a pretty busy diagram, but I'll walk you through it.
00:16:18.02 At the top, this is merely a depiction of the VSV genome and the 5 genes N, P, M, G,
00:16:24.14 L. And what you can see is, for each one of the lineages ,the 4 lineages that saw only,
00:16:30.10 in this case, HeLa cells... those are cancer-derived cells that originally came from Henrietta
00:16:35.22 Lacks a long time ago, and these were harnessed as an immortalized cell line that people use
00:16:41.08 and a lot of studies beyond simply virus studies... but these cancer-derived cells provided a
00:16:47.07 new challenge for VSV in this experiment, and each one of the points, here, on the diagram,
00:16:52.19 are showing where these lineages changed in their genetic material relative to the ancestor
00:16:59.12 after the experiment took place.
00:17:01.18 In this way, we can catalogue, what are the mutations that arose, and which ones fixed
00:17:06.10 through natural selection, to let these lineages improve in their environment?
00:17:12.00 We also did an exp... in this experiment a challenge where the viruses had to not only
00:17:15.23 evolve on HeLa cells, but, in an alternating fashion, they had to enter into a non-cancer-derived
00:17:22.12 cell type, abbreviated as MDCK, and in this way they had to become adapted to both HeLa
00:17:28.12 cells as well as these non-cancer cells.
00:17:31.18 And you'll see that we also catalogued their genetic changes through time.
00:17:35.24 And this has a great deal of variety, even within each treatment, for the mutations that
00:17:41.15 fixed according to each lineage.
00:17:44.02 One can also catalogue the exact position where each one of these mutations took place.
00:17:48.12 Let me highlight one more thing before I move on, and that is, really, these virus populations,
00:17:55.08 after this experiment, are not carbon copies of one another.
00:17:58.19 So, there are many places where we do see that they underwent the same mutational change
00:18:04.08 at exactly the same place, and that must be evidence of some beneficial mutation coming
00:18:10.01 in and fixing in these lineages.
00:18:12.15 And yet, in some genes, they underwent different mutations from even the same populations in
00:18:17.21 the same treatment, so this indicates that there can be other genetic solutions to the
00:18:22.22 same environmental problem in a study like this.
00:18:26.06 Keep this in mind as we go on and look at a subsequent experiment that challenged the
00:18:31.02 ability of these viruses to evolve and infect yet new hosts to test, what is their emergence
00:18:37.15 capacity?
00:18:39.01 Simply remember that we lumped them together as specialists, having seen only one constant
00:18:44.05 host hype, or generalists, that were selected to see two types, and yet the lineages are
00:18:49.10 not carbon copies of one another when they're drawn from each treatment.
00:18:53.16 Here, we wanted to ask a very fundamental question that's really at the root of what
00:18:58.15 lets emergence occur.
00:19:00.11 So, a popular idea is that, if some pathogen has seen multiple hosts in the past, it's
00:19:07.02 somehow groomed through adaptation to be generalized enough that it will successfully enter and
00:19:13.16 infect a new host when it sees it just randomly through encountering it in nature.
00:19:19.08 That's because adaptation has primed that pathogen to be good at growing in multiple
00:19:23.24 hosts and, through correlated response, it just might grow very well in a new host such
00:19:28.13 as humans.
00:19:29.13 So, here, I'm depicting a picture of Henrietta Lacks, as the... ultimately, the person who
00:19:34.16 gave rise to these HeLa cells that we used in this experiment, and we asked, whether
00:19:39.13 viruses that evolved strictly on HeLa cells, are they going to be good at growing on a
00:19:45.06 variety of challenge hosts that we purchased?
00:19:48.20 Or are we going to fit with this prediction that selected generalists were pre-adapted
00:19:55.06 in some way to perform well on these new hosts and they should be the ones that we would
00:20:00.00 fear as typical of a successful emerging pathogen, something that's groomed to grow on multiple
00:20:06.01 hosts and will grow well on a challenge host when it encounters it?
00:20:10.24 To go to the data from an earlier paper, this is pretty well supported by our study, that,
00:20:17.15 yes, selected generalists emerge or they shift hosts easier.
00:20:21.16 So, this diagram is showing, what is the sheer reproductive capacity of each of these virus
00:20:28.04 lineages, indicated by each point, relative to its ability to grow in the environment
00:20:33.19 that it was previously evolved on?
00:20:35.22 So, this gives an indication of... relative to its ordinary reproductive capacity, is
00:20:41.16 it any better or equally good at growing on a new challenge host, relative to the host
00:20:46.18 that it saw prior to adaptation?
00:20:49.13 And you'll see that all the blue points are well below the zero line.
00:20:53.19 That means that these specialist viruses from our study, they can grow on this first challenge
00:20:59.16 host I'm indicating, that came from monkey cells, but they grow pretty poorly compared
00:21:04.02 to their capacity to grow on the HeLa cells that came from Henrietta Lacks, whereas the
00:21:09.06 selected generalists, they saw both host types in our prior experiment -- one happened to
00:21:15.05 be cancer-derived, one happened to be non-cancer-derived -- but those were different enough cell types
00:21:20.09 that have provided a challenge to adapt to two things simultaneously.
00:21:25.03 And you'll see that, on this challenge host, those selected generalists actually did a
00:21:29.07 better job at growing on a challenge host that was just randomly chosen and presented
00:21:34.05 to them.
00:21:35.16 All four of those triangles are very close to the zero line.
00:21:38.22 So, in summary, one could say that, in this first line of evidence, on the monkey cells,
00:21:45.00 there's both a higher mean, on average, and lesser variance across the populations drawn
00:21:50.15 from each treatment for the selected generalist to do better.
00:21:53.24 Now, if you look at all four challenge hosts, there's an amazing ability for the data to
00:22:00.21 look highly similar, no matter what the challenge host was that we randomly entered into this
00:22:05.18 experiment using.
00:22:07.02 And, to me, that's fascinating, because it indicates that there's hardly any of what
00:22:11.22 one would call genotype-by-environment interaction.
00:22:14.22 This must be due to the capacity of these viruses to just simply grow on something new,
00:22:20.17 and it's not really the interaction with that new thing, it's just that they can grow better
00:22:25.02 on something that they've been challenged to infect.
00:22:27.07 So, this provides nice evidence in four randomly chosen challenges that selected generalists
00:22:33.21 can grow much better on a new host that you present them with, and this gives us a little
00:22:38.13 more insight at what could be the root of the emergence problem.
00:22:42.16 But I haven't really told you why -- why is this happening?
00:22:48.10 Why is it that these selected generalists actually emerge easier at a mechanistic level?
00:22:53.20 Here, we've looked at the ability, the innate immune ability, of cell types, and whether
00:22:59.24 selected generalists were keying in on this line of immunity and navigating their way
00:23:05.24 through it, and if they have a generalized ability to do that, and that should carry
00:23:10.22 over to other challenge types, even though that challenge type would be drawn from a
00:23:15.08 different species.
00:23:16.16 So, this is a very detailed diagram, but it's showing some of the inner workings at the
00:23:21.11 cellular level of something that you're born with.
00:23:24.22 This is the innate immune capacity of your cells that, when they see an invader, like
00:23:29.22 a virus, that they will be able to undergo a cascade of events at the cellular level
00:23:35.13 that gives them protection against that virus infection.
00:23:39.00 And, interestingly, the signals can go out to cells that are nearby in the tissue neighborhood
00:23:45.14 to prime them to be better protected against that virus, before the virus even is able
00:23:51.08 to replicate enough to get to those cell types.
00:23:53.24 Now, this is a wonderful ability, to be immune to a pathogen, that you should remember this
00:24:00.00 is your innate immunity.
00:24:02.13 Adaptive immunity, which people are much more familiar with, is something that is occurring
00:24:06.08 much longer-term, and it takes weeks or even longer that you see a pathogen and you mount
00:24:11.00 an immune response to the its uniqueness.
00:24:13.07 Here, this is just a generalized thing that controls pathogen infections.
00:24:18.06 So, before I move on, I'll say that the VSV M protein, or the matrix protein, is known
00:24:25.09 to be the thing that interacts with the capacity of a cell to produce its anti-immune response
00:24:31.11 to virus infection, especially interferon.
00:24:34.06 So, ordinarily, this cell is going to be producing interferon as one of these key chemicals that
00:24:39.09 protects it, and signals go out and interferon production occurs in other cells in the tissue
00:24:44.18 to protect them, but VSV, as a virus, can infect a cell and down-regulate that response.
00:24:53.05 And that helps us even explain how we even did the prior experiment.
00:24:56.19 VSV has a great capacity, just as a virus, to grow in a variety of cell types, because
00:25:03.18 it can regulate this response.
00:25:06.08 However, it could be that viruses like VSV are highly generalized in moving between hosts
00:25:14.18 because they properly regulate that immunity cascade.
00:25:19.15 So, without very many details, this is a hypothetical idea of how this can occur, and what one should
00:25:25.24 expect.
00:25:26.24 So, let's imagine that the prior selection history of some virus or other pathogen, this
00:25:32.19 is relating its fitness, due to that prior evolution, in terms of whether it saw host
00:25:38.22 types that are of low or high innate immunity.
00:25:41.23 So, in our prior experiment, I highlighted in blue how these specialist viruses perform
00:25:48.12 very well on HeLa cells, but I didn't tell you one key bit of information about a lot
00:25:54.15 of cancer cells, including HeLa cells.
00:25:57.03 They have very low or completely absent innate immunity.
00:26:01.00 So, what happened in that experiment, probably, is that the lineages of viruses evolved to
00:26:06.03 infect a cell type where they didn't really have to worry at all about innate immunity
00:26:10.17 as a challenge in infecting and growing in the cell type.
00:26:14.00 So, this probably led to de-evolution, or the removal of the capacity for those viruses
00:26:20.09 to control innate immunity.
00:26:22.00 They just simply didn't need it.
00:26:24.05 And then, when you challenged them to grow on a new host type, they are very handicapped
00:26:28.19 in doing so because they don't have the capacity to track the innate immunity functions within
00:26:33.08 a cell.
00:26:34.09 Whereas, viruses could see, necessarily, in our experiment, both high and low innate immunity,
00:26:40.24 because we used cancer-derived as well as non-cancer-derived cells, so they remained
00:26:45.13 capable of navigating through both cell types, and when they see a new cell type they can
00:26:50.15 hit the ground running.
00:26:52.06 So, importantly, one can think of both alternating hosts as, necessarily, in our experiment,
00:26:59.17 keeping this capacity, but it also could have been, and we've done work like this... if
00:27:04.08 you take virus lineages and you grow them only on high innate immunity hosts, you get
00:27:09.16 a very similar capacity for them to maintain strong growth regardless of cell type.
00:27:15.15 So, we have both good news and bad news in predicting emergence.
00:27:19.21 We have the ability for selected generalists to key in on innate cell function and navigate
00:27:25.09 multiple cell types, and you'd expect them to emerge, but they don't have to do that.
00:27:30.03 They could still successfully emerge through a correlated response.
00:27:34.00 So, the next question I want to cover is whether the environmental change that is presented
00:27:40.17 to viruses either fosters or constrains their adaptation.
00:27:44.05 So, now, this is a similar diagram that I showed you before, but note that now I'm including
00:27:48.17 a different kind of a challenge.
00:27:50.19 Here's where the lineage sees pretty much a stochastic set of environments through time.
00:27:56.03 In other words, it's moving from environment to environment, but there doesn't seem to
00:28:00.13 be any pattern to what that... what those environments present, right?
00:28:04.10 So, these are shown as separate colors in this diagram to illustrate how some virus
00:28:09.10 lineages might have to navigate through very different environments, and one can create
00:28:14.02 an experiment that says, well, will these champions of adaptations still be able to
00:28:19.12 successfully navigate through such a complex set of environments and become generalized?
00:28:24.20 Or is this just simply too much and, even in champions of adaptation like RNA viruses,
00:28:30.08 they'll be constrained and unable to do this?
00:28:34.04 This actually relates in some way to certain models that come from climate change, where
00:28:40.02 the prediction is... really, the fundamental problem for evolving lineages in climate change
00:28:46.05 is that stochasticity of the environment becomes more important.
00:28:50.07 The environment simply becomes more variable through time and it will be harder for lineages
00:28:54.21 to track those changes.
00:28:56.23 Well, it should be interesting to see whether viruses can successfully do this, because,
00:29:02.10 if they cannot, then this bodes pretty bad news for other organisms that have a much
00:29:07.12 slower and reduced capacity to evolve in the face of environmental challenges.
00:29:12.11 So, let's see what happened.
00:29:14.20 one can easily construct an experiment like this, but, rather than creating host challenges
00:29:20.04 through time, let's think a little bit more about those climate change models and the
00:29:24.01 thing that we'll manipulate is temperature through time.
00:29:26.14 So, in this diagram I'm showing four different treatment groups that were created in an experiment,
00:29:31.24 where 37 degrees Celsius is the upper limit, or pretty much the ordinary temperature for
00:29:37.01 replication that we use in the laboratory for VSV; 29 degrees Celsius is a lower temperature,
00:29:44.00 where they can still grow but it's much lower than 37 C, 8 degrees lower; and then we have
00:29:49.17 alternating lineages that will see these two environments in a flip-flopping fashion; and
00:29:55.12 then we include this fourth treatment, this is really the intriguing one.
00:29:58.21 If you take that 8-degree window and you go into the laboratory and you challenge the
00:30:03.06 viruses to grow at any temperature in the 8-degree window that you randomly choose on
00:30:07.10 that day, it will create a very stochastic environment through time.
00:30:12.00 And here we want to know, across generations, especially 100 generations, is there any differing
00:30:17.09 capacity of these viruses to evolve well in the face of this challenge?
00:30:22.06 So, we can go immediately to the data that came from this experiment.
00:30:26.04 And the way this graph works is it shows you, what is the fitness after 100 generations
00:30:30.24 for each one of these lineages, at each edge of the niche space?
00:30:35.02 So, it's plotted, what is their fitness at 37 C versus their fitness at 29 C?
00:30:40.24 And the intersection of those points leads to each point on the graph.
00:30:44.11 So, you'll see that the lineages that evolved in a constant high-temperature environment
00:30:49.00 improved in that environment -- in other words, all their data are to the right and above
00:30:55.05 the dashed lines on this figure.
00:30:57.03 They've improved both in terms of the environmental challenge they saw -- 37 C -- as well as 29
00:31:03.21 C, which was the other environment that was constant in this experiment.
00:31:07.02 That's evidence of correlated selection -- you improve in one environment and it also allows
00:31:12.05 you to improve in another environment that you haven't seen.
00:31:15.01 The same thing occurred for the lineages that saw only 29 C as the challenge.
00:31:20.08 Interestingly, in green, we have an alternating environment, where populations improved, in
00:31:27.09 some cases more than populations that saw only a constant environment.
00:31:32.10 And that's intriguing because it shows that populations can improve even though they see
00:31:36.11 the challenge only half the time as their counterparts.
00:31:40.08 It must be that the genetics that underlies this, which we've shown in papers that I won't
00:31:44.00 present today, is that different mutations are responsible for this improvement in an
00:31:48.09 alternating environment versus a constant one, but, in both cases, you can have improvement
00:31:53.03 relative to the ancestor.
00:31:55.08 Most intriguing in this data set is shown in purple, where all of those purple points
00:31:59.23 and lines are nestled right near the intersection of the dashed lines, which show the ancestral
00:32:05.10 values.
00:32:06.10 This means that the random treatment in the pop... in the experimental evolution study...
00:32:12.09 these lineages did not improve any more than the ancestral performance.
00:32:17.13 In other words, the stochasticity of the environment was too much for them to deal with, and that's
00:32:22.11 bad news in terms of these champions of adaptation.
00:32:26.08 If they can't handle stochastic environments, then that bodes ill for more complex organisms
00:32:32.20 that have much slower adaptive and evolutionary capacity -- we wouldn't expect them to thrive
00:32:38.07 either, or to improve in fitness, when seeing stochastic change.
00:32:43.16 This is pointed to in the diagram in terms of the intersection of the points, and all
00:32:47.20 those purple points nestled near the dashed lines and their intercept, as indicative of
00:32:53.22 adaptive constraint.
00:32:56.20 The next question will be, do viruses evolve survival reproduction trade-offs that we observe
00:33:01.16 in cellular life?
00:33:03.00 Here, we want to examine whether the capacity to adapt in one means, and that is to produce
00:33:09.13 progeny, is something that detracts from the capacity to merely survive in the environment
00:33:15.02 when it poses a challenge.
00:33:16.02 We've seen in cellular systems that you can't have your cake and eat it too, in terms of
00:33:22.02 these two challenges improving through time, that you can either invest in survival or
00:33:26.21 reproduction, but often you have an inability to improve in both simultaneously.
00:33:31.18 Does this carry over to the virus world is an intriguing question.
00:33:35.18 We addressed it first in a phage called phi-6 that infects a bacterium known as Pseudomonas...
00:33:42.01 Pseudomonas syringae.
00:33:43.10 So, this bacterium is important in plant pathology -- it causes plant disease -- but in the laboratory
00:33:50.10 we merely use it to grow the phage as a resource, to examine how well does the phage evolve
00:33:56.12 in environments in the laboratory.
00:33:58.06 So, this is an RNA virus, so it has the capacity to undergo error rate at a high rate, and
00:34:05.04 this allows a lot of mutation and rapid change through time, and it has a very typical infection
00:34:10.11 cycle, where it infects a cell of the bacterium, bursts the cell for the progeny to be released,
00:34:16.11 and then they go on and infect more cells.
00:34:18.05 That's a lytic phase replication cycle.
00:34:21.23 This picture is showing how the virus is able to first infect cells, because the cells have
00:34:28.24 the structures that allow them to adhere to leaf surfaces and, in normal wild conditions
00:34:34.22 they would move across the leaf and enter into the plant, in order to do infection of
00:34:39.10 the plant.
00:34:40.10 So, through time, these viruses have evolved the ability to use those structures as the
00:34:45.08 thing that they attach to, through protein binding, to get into the cell.
00:34:49.13 And that's what's shown in the diagram.
00:34:51.24 So, one can first begin by examining a reaction norm, or just simply the capacity for phi-6
00:34:59.03 to grow under environmental challenges in the laboratory, and, even though those challenges
00:35:03.14 can be amazingly brief, only five minutes long in terms of heat shock, this diagram
00:35:09.14 is showing how the survival of a virus population of phi-6, relative to different heat shock
00:35:17.02 temperatures, a high degree of mortality starts to kick in well above the normal incubation
00:35:22.14 temperature in the laboratory of 25 C. When you get out to values greater than 40 C, you
00:35:29.07 find that this is highly impactful and deleterious to the viruses and their ability to thrive.
00:35:34.20 So, this is indicating how, in the absence of anything else, you can take this virus,
00:35:40.13 expose it to high heat, and, if the heat is high enough, it leads to a high degree of
00:35:45.04 mortality in the virus population.
00:35:47.17 Key in on both 45 and 50 C, where these are environments that we've manip... manipulated
00:35:53.22 in the laboratory to examine, how do viruses deal with heat shocks through time if they
00:35:59.18 see them, and can they key in on this high heat that leads to high mortality and become
00:36:05.16 better adapted to thriving in the face of heat shock?
00:36:10.01 This is a diagram from a recent paper, where it's simply showing you a typical experimental
00:36:13.22 design for a study like this.
00:36:16.03 If you just take the virus, such as in a test tube, in the absence of any cells, and you
00:36:21.03 put it in a heating block so that it'll be challenged with five minutes of high heat,
00:36:26.04 you can then take the viruses and grow them under normal low-heat conditions, where they
00:36:30.13 can replicate in the presence of bacteria, gather all that up, remove the cells, and
00:36:36.24 keep churning them through the experiment.
00:36:39.10 In this way, we're not worried about whether they can, say, co-evolve with the host bacteria;
00:36:45.14 we're mostly keying in on the thing that causes high mortality -- the heat shock.
00:36:50.05 Can they key in on that and become better at thriving and improve this value, which
00:36:56.19 shows their very strong mortality that they suffer under high-heat environments?
00:37:02.01 Going quickly to the data from a paper where we did such an experiment, we find that thermal
00:37:07.16 tolerance, or heat shock selection, can readily occur in these viruses, and this is indicative
00:37:13.22 of something that we would call environmental robustness.
00:37:16.13 So, what is the ability of some population to thrive across different environments, and
00:37:22.14 maintain high fitness?
00:37:24.02 You'll see that the lineages shown in red, those that came out of an experiment where
00:37:28.09 this virus saw intermittent heat shocks at 50 C, these lineages improved way out at this
00:37:36.07 temperature, and you'll see this through a statistical result, that they do grow better
00:37:41.20 than their ancestral virus at that very high temperature.
00:37:45.05 Now, it's not like they have absolute capacity to shrug off that heat shock, but they do
00:37:50.07 have greater capacity to do so.
00:37:52.16 And, interestingly, you can see how there's a huge effect at the lower temperatures, which
00:37:57.15 ordinarily are degrading the wild type or unevolved virus, and now these lineages that
00:38:03.04 saw only 50 C have a great capacity to thrive at very, very warm temperatures and including
00:38:10.01 the highest temperature that they saw in the experiment.
00:38:13.17 How does this occur?
00:38:15.10 We've done several experiments of this type and always, for this virus, the same key mutation
00:38:21.06 is the first one and the most important one that leads to thermal tolerance evolving.
00:38:28.01 phi-6 has a genome that's split up into three different segments called large, medium, and
00:38:33.05 small.
00:38:34.05 In this diagram, it below shows that we know what all the genes are and we know basically
00:38:37.12 what their functions.
00:38:39.04 And here we have a diagram, a cut-through, of the virus body plan that shows you that
00:38:43.20 that... all that nucleic acid is at the center of the virus and it's surrounded by a protein
00:38:48.23 shell.
00:38:49.23 But, uhh... cystoviruses -- this is the family that phi-6 belongs to -- they're are a little
00:38:54.20 different than other bacteriophages in that they have a lipid coat around the entire shell.
00:39:00.12 So, it's a pretty elaborate body plan for a phage, but I really only want you to understand
00:39:06.01 that the key mutation that provides thermal tolerance always seems to arise first on the
00:39:11.06 small segment, and it always seems to arrive in this lysin gene, which is we... going to
00:39:16.24 be responsible for virus particles both getting in and out of the cell, and it is always the
00:39:23.00 same mutation, V207F.
00:39:26.04 It's an amino acid substitution that I'll talk about further.
00:39:29.24 V207F seems to be the key mutation that always allows the viruses to evolve thermal tolerance.
00:39:36.13 And, mechanistically, this makes sense, because when you look at the structure of this lysin
00:39:42.03 protein, a very important enzyme, phenylalanine as an amino acid substitution fills a hydrophobic
00:39:49.06 pocket, and this makes the protein more stable under high heat.
00:39:53.22 So, this is only one mutation coming in but it has profound significance for the thing
00:39:59.10 that is causing high mortality in the virus populations.
00:40:03.03 It's a very simple explanation of how a single amino acid substitution can lead to a profound
00:40:09.15 ability to thrive under a key environmental challenge.
00:40:12.19 So, now, I will talk about how the reproduction is affected for this virus -- even though
00:40:20.15 the key mutation allowed better survival, it's detracting from reproduction.
00:40:25.00 So, if we look at, how does this V207F mutant thrive in an ordinary environment, 25 C, and
00:40:33.03 its ability to grow on bacteria, this diagram is first showing how the plaques... in other
00:40:38.22 words, when you take a virus and you grow it on a bacterial lawn, which is the background
00:40:44.15 in this diagram in white, each one of the particles, if they hit the lawn independently,
00:40:49.18 they'll infect a cell and the progeny will exit that cell, infect neighboring cells,
00:40:54.23 and eventually you'll get this hole in the bacterial lawn called a plaque.
00:40:58.17 Well, something interesting happens when you look at the morphology of the plaques of the
00:41:03.03 wild type virus, which is heat-sensitive, versus this V207F mutant that is heat-tolerant.
00:41:09.19 In all cases, the V207F mutant makes this weird-looking plaque that has a bull's-eye...
00:41:16.11 bull's-eye morphology to it.
00:41:18.09 So, that must be that cells missed being infected and killed as that plaque was produced on
00:41:25.10 the lawn, otherwise it wouldn't have that grayish appearance.
00:41:28.02 In other words, it is not as effective at killing cells even though it is thermal tolerant.
00:41:34.07 That's shown in the bar graph, here, where the selection coefficient or "little s" that
00:41:39.13 is associated with this one mutation has a huge deleterious value under normal growth
00:41:45.20 conditions.
00:41:46.21 The wild-type relative to itself, of course, grows equally well so we give it a value of
00:41:52.03 1, whereas the value for the thermal-tolerant mutant is a value much, much lower than 1,
00:41:58.12 and has a negative selection coefficient of 0.25.
00:42:03.00 In comparison to the data I showed you earlier, it's very evident that a life history trade-off
00:42:08.04 is occurring in this virus.
00:42:09.17 In other words, it can either invest in better survival, but the problem is this leads to
00:42:16.17 lower reproduction.
00:42:18.11 This is echoing something that we see in cellular systems, with the investment in either survival
00:42:23.05 or reproduction, but not both at the same time occurring simultaneously.
00:42:29.07 It also relates to an earlier study, where the researchers found that if you just randomly
00:42:35.02 take viruses that can infect a different bacterium -- E. coli -- and you look at what is their
00:42:40.13 mortality rate versus their multiplication rate, and you plot that on the same graph,
00:42:45.17 there's a pretty amazing relationship for these viruses that they produced in their
00:42:49.20 study or used in their study to show that these are highly correlated traits to one
00:42:55.13 another.
00:42:56.13 So, either these viruses that they studied grew well and survived poorly, or had a high
00:43:01.09 mortality rate, or they grew poorly and survived better.
00:43:05.10 So, it shows that if you just look at viruses from the natural environment and you look
00:43:11.13 at their relationship for survival versus reproduction, they show a big difference,
00:43:16.22 and they fall along this line.
00:43:18.24 You can think of our experiment as having taken any one virus on this line, and can
00:43:23.17 you move it up or down the line through an experimental evolution study, and that's exactly
00:43:27.24 what we did.
00:43:28.24 So, the survival reproduction trade-off holds, and you can move them up and down the line
00:43:33.24 if you vary the environment in the right way.
00:43:36.16 So, the bull's-eye plaque that I showed you before is a pretty strange morphology.
00:43:43.19 And there's actually... even though people have used old microbiology methods to the
00:43:47.16 current day, the ability to visualize plaques is something that has dated back to at least
00:43:52.23 the 1940s, so it's an old method, and yet we actually don't know very much mathematically.
00:43:58.15 If you construct a model about, how does a plaque form, this is still a pretty big challenge
00:44:03.04 to mathematical biologists.
00:44:05.05 The three-dimensionality that this plaque is growing in, on an agar surface, is something
00:44:10.07 that... it's very hard to describe mathematically.
00:44:12.13 And, especially if one looks through a time-lapse film of these types of bull's-eye plaques
00:44:19.02 forming, this is a very strange morphology that it's hard to understand how some cells
00:44:24.19 are killed initially, and then there's a lot of cells that do not get killed, and then...
00:44:29.10 and then more cells get killed, and so on, to lead to such a complex morphology.
00:44:33.13 I would say that this is still an ongoing challenge to simply describe how do plaques
00:44:38.07 form, mathematically and mechanistically.
00:44:40.22 In this example from our experiment, even one mutational change can lead to very different
00:44:46.00 morphology that provides an even bigger challenge to describe.
00:44:50.08 So, now, we can think of the viruses and the way that they encounter challenges in the
00:44:57.12 natural world as, yes, they have an amazing capacity to see challenges and overcome them.
00:45:05.01 So, we do fear emergence of viruses as something that will continue to be a challenge for humans,
00:45:11.23 domesticated species, conserving endangered species... all of these realms are threatened
00:45:17.24 by the emergence of viruses coming in and doing destruction.
00:45:21.14 However, there are certain environments where these champions of adaptation simply cannot
00:45:26.11 make it.
00:45:27.12 So, consistent with certain climate change...
00:45:29.14 climate change models, we have environmental change through time as something that can
00:45:33.16 constrain virus evolution, and some of the fundamental trade-offs that we see in cellular
00:45:38.13 systems, especially survival versus reproduction, carries over even into organisms that don't
00:45:45.07 undergo metabolism -- the viruses -- so it's not just they're shunting energy into one
00:45:49.16 thing or another.
00:45:51.01 It shows you more of a fundamental divide in the biological world of, can you invest
00:45:55.20 in survival versus reproduction, and get away with a co-investment in both of them?
00:46:00.07 And it seems like that's not the case.
00:46:02.10 So, I'd like to end by acknowledging the people who did this work.
00:46:06.11 I keyed in on a lot of the work done by my current lab group, as well as past lab members
00:46:10.24 who I've had the pleasure of working with.
00:46:12.20 I've had fantastic mentors and collaborators all over the world.
00:46:17.16 And I have to thank them deeply for their dedication to the experiments to present the
00:46:21.13 data that wound up in our papers.
00:46:23.22 I can also thank the funders for the work, NSF and its programs such as the BEACON Center
00:46:31.09 for experimental evolution, as well as NIH, Yale University, and nonprofits such as the
00:46:38.02 Project High Hopes Foundation have provided key funds for all the work that I showed you
00:46:43.15 today.
Host Evolution
Concepts: Molecular arms races between primate and viral genomes, host evolution, host-virus interactions, natural selection of mutations, and PKR signaling evolution across primates
Please watch original video from time 00:00 to 21:10.
- Duration: 21:10
- Downloads
- Subtitles
- Transcript
00:00:00.28 Hello.
00:00:02.01 My name is Harmit Malik,
00:00:03.11 and I'm an evolutionary geneticist
00:00:05.03 studying the evolution of viruses and host genomes
00:00:07.28 at the Fred Hutchinson Cancer Research Center.
00:00:10.15 Today, I'm actually going to tell you
00:00:11.28 about molecular arms races between primate
00:00:14.09 and viral genomes
00:00:15.25 and how we aim to understand
00:00:18.01 the evolutionary rules that take place
00:00:20.02 between these viruses and hosts
00:00:21.24 and what that will tell us about,
00:00:23.13 not just the evolution of ourselves and viruses,
00:00:26.11 but also to design therapeutics interventions
00:00:28.23 to allow us to designed better strategies
00:00:31.06 that are going to be effective against viruses.
00:00:33.22 The work in the field of molecular arms races
00:00:36.25 is really inspired by
00:00:39.10 the character the Red Queen that was introduced to us
00:00:41.25 by Lewis Carroll in his book "Through the Looking Glass",
00:00:44.20 and the Red Queen tells Alice
00:00:46.29 in this sort of nice book
00:00:50.07 that it takes all the running you can do
00:00:52.11 to keep in the same place.
00:00:54.01 Very much the same idea
00:00:55.27 was adopted by the evolutionary biologist
00:00:58.01 Leigh Van Valen,
00:00:59.23 as the Red Queen hypothesis,
00:01:01.28 and he argued that in a system
00:01:04.00 where two entities are constantly competing
00:01:05.22 with each other in this sort of battle
00:01:07.22 for evolutionary supremacy,
00:01:09.15 the only way for this battle to be resolved
00:01:12.08 is just for one party to temporarily win
00:01:14.29 before the other party catches up,
00:01:16.26 and this requires both of these parties
00:01:19.01 to be really running as fast as they can
00:01:21.04 with this really rapid evolutionary signature,
00:01:23.09 formalized as the Red Queen Hypothesis
00:01:25.15 that's been used to invoke
00:01:27.21 all kinds of very important principles
00:01:29.19 in evolutionary biology,
00:01:31.13 including the existence of sex
00:01:33.16 and why we actually evolved
00:01:35.21 to be sexual creatures in the first place.
00:01:38.22 So, if you consider a host-virus interaction,
00:01:41.06 this is an interaction
00:01:43.00 that screams out genetic conflict.
00:01:44.23 This is what we refer to
00:01:46.13 as the usual suspects.
00:01:48.00 It doesn't take a lot of imagination
00:01:49.13 to understand that what is in the best interest of the virus
00:01:52.10 will not always be in the best interest of the host.
00:01:55.03 So, in this cartoon example,
00:01:56.25 you can see that we've got two states described here,
00:01:59.24 you've got the host that is binding the virus
00:02:02.08 on one side,
00:02:03.25 and the virus that has evolved a mutation
00:02:06.03 to evolve away from that recognition
00:02:07.28 by the host immune system.
00:02:09.17 What you'll actually appreciate
00:02:11.20 is that these state transitions,
00:02:13.11 between one state and the other,
00:02:14.28 are really profound but very simple
00:02:16.26 from a mechanistic standpoint.
00:02:18.25 What it might take is just a single amino acid mutation
00:02:21.10 for the virus to gain one step ahead
00:02:23.19 in this battle for evolutionary supremacy.
00:02:26.09 So, the important take-home message
00:02:27.25 from this kind of slide is,
00:02:29.14 one party is always losing
00:02:31.22 this high-stakes evolutionary battle.
00:02:33.13 On the left-hand side
00:02:34.28 you can see that the host is winning,
00:02:36.18 because it is recognizing a viral protein.
00:02:38.14 On the right-hand side
00:02:39.23 you can see that the host is losing,
00:02:41.13 because the virus has acquired the right mutation
00:02:43.22 that allows it to evade detection by the immune system,
00:02:46.15 which basically means that there's never going to be
00:02:49.10 a perfect equilibrium between these two states.
00:02:51.10 Over the course of evolution,
00:02:53.01 and even the course of a single infection in a person,
00:02:56.02 the immune system and the virus
00:02:58.26 are basically locked in this arms race
00:03:01.03 of very rapid evolution
00:03:03.09 and, because one party is always losing,
00:03:05.03 there's always going to be an evolutionary advantage
00:03:07.06 to be gained by innovation.
00:03:09.06 Now, we're going to actually talk about
00:03:11.04 two types of innovation today.
00:03:12.18 In the first part of my talk,
00:03:14.04 which is focused exclusively on how hosts evolve
00:03:16.28 in the face of viral challenges,
00:03:18.28 we're going to specify innovation
00:03:21.10 in protein coding genes,
00:03:23.03 and so, if you consider
00:03:25.05 what a protein coding gene arbitrarily looks like,
00:03:28.11 it's this sort of sequence that I've indicated here,
00:03:31.02 where we've got three triplets, three codons,
00:03:34.00 that specify three amino acids
00:03:35.25 that will be incorporated into the protein
00:03:37.17 that is produced from this gene.
00:03:39.15 Now, you can see on this side,
00:03:41.15 you have a mutation
00:03:43.17 that does not alter the amino acid being encoded.
00:03:45.23 We refer to these as silent or synonymous changes,
00:03:48.27 because from a very sort of rough approximation
00:03:51.17 natural selection is really acting on
00:03:54.01 the protein coding sequences,
00:03:55.19 and here, because the protein coding sequence
00:03:57.10 has not altered, we refer to these as
00:03:59.22 silent or synonymous changes.
00:04:01.14 In contrast, you can see here we have,
00:04:03.21 again, a single amino acid mutation,
00:04:05.28 which has altered one of the amino acids
00:04:08.13 that's being encoded,
00:04:10.05 so-called non-synonymous or replacement changes.
00:04:12.21 Now, both of these
00:04:14.25 are sort of equal likelihood mutations. Y
00:04:16.21 ou can actually have a synonymous mutation
00:04:18.16 or a non-synonymous mutation,
00:04:20.08 but you can appreciate that, based on the genetic code,
00:04:22.18 you're much more likely
00:04:24.22 to see an amino acid-altering mutation,
00:04:26.18 just by random chance alone.
00:04:29.18 So, consider the sort of situation
00:04:32.19 where you actually had a gene,
00:04:34.23 we refer to these as pseudogenes,
00:04:36.29 that at some point in their evolutionary history
00:04:39.02 encoded for a particular protein.
00:04:41.19 Now, if you consider this gene
00:04:44.09 now in its current degenerate form,
00:04:46.22 let's say built from the chimpanzee genome
00:04:48.16 versus the human genome,
00:04:50.09 and we were to just roughly calculate
00:04:52.07 the number of synonymous changes
00:04:54.15 versus replacement changes,
00:04:56.17 we have to correct for the fact
00:04:58.10 that there are many more possible replacement changes,
00:05:01.01 so when you normalize for that correction
00:05:03.02 you will find that, because this gene no longer codes
00:05:05.18 for a protein,
00:05:07.09 the rate of synonymous changes
00:05:08.24 and the rate of replacement changes
00:05:10.15 are roughly equal,
00:05:11.25 and that's because selection has stopped worrying
00:05:14.13 about this part of the genome
00:05:16.02 in terms of its protein-coding capacity.
00:05:17.24 It has tolerated both mutations,
00:05:19.22 and they roughly go to fixation
00:05:21.20 in a fairly random fashion.
00:05:23.15 Now, for most genes in the genome,
00:05:25.08 you do care about the final product being produced,
00:05:27.16 which is the amino acid sequence
00:05:29.19 of the resulting protein.
00:05:31.07 So, here I have this hypothetical example
00:05:33.14 where you have a protein-coding gene
00:05:36.14 that is basically representing these triplets of codons,
00:05:39.12 and what you'll see is there's a lot more blue changes,
00:05:42.11 or non-amino acid altering or silent changes,
00:05:46.23 and very rarely do you see something
00:05:48.22 which looks like a replacement
00:05:51.13 or a non-synonymous change.
00:05:53.09 The net result is that,
00:05:55.00 regardless of all of this change at the nucleotide level,
00:05:57.16 the amino acid sequence remains STEVE,
00:06:00.01 because STEVE is really what is being selected for
00:06:03.00 by evolution.
00:06:04.11 Very rarely do you see a deviation
00:06:06.13 from this optimal amino acid sequence.
00:06:08.16 For instance, we can see SiEVE coming in,
00:06:10.29 in terms of this sort of grammar.
00:06:13.00 The net result is not that
00:06:16.20 we should infer that mutation has now stopped
00:06:19.06 hitting the replacement sites.
00:06:20.27 What we infer from this is,
00:06:22.28 because mutation has introduced changes
00:06:24.22 in both replacement and synonymous positions,
00:06:27.19 the fact that we don't see replacement changes
00:06:30.01 over the course of evolution
00:06:31.26 is an indication that natural selection
00:06:34.03 acted upon these changes,
00:06:35.21 deemed them deleterious,
00:06:37.18 and removed them from the population
00:06:39.10 before they had a chance to really spread
00:06:41.28 in the population,
00:06:43.21 which means mutations is not really causing
00:06:45.19 this bias between the blue and the red changes.
00:06:48.06 It's actually natural selection,
00:06:50.02 and, more specifically, purifying selection,
00:06:52.06 that is acting to purify the population
00:06:54.16 from these presumed deleterious mutations.
00:06:56.26 The net result is,
00:06:58.21 if you were to now compare the rate of
00:07:01.02 synonymous and replacement changes,
00:07:02.29 we will find that the rate of replacement changes
00:07:04.25 is actually much lower than synonymous changes,
00:07:07.26 regardless of the fact that both of these changes
00:07:10.00 were introduced in roughly the same proportion.
00:07:13.11 My lab is actually interested in the other
00:07:15.00 class of genes that emerges
00:07:16.20 from these kinds of analyses.
00:07:18.05 Here again, now, we have a triplet code of sequences
00:07:21.03 that encodes for my name in amino acid code,
00:07:24.18 and what we will see when we compare
00:07:26.26 across this sequence
00:07:28.27 is that there are a lot more red changes
00:07:30.27 than blue changes,
00:07:32.09 in fact a lot more red changes than what you'd expect,
00:07:34.20 even by chance alone.
00:07:36.19 It's in fact easier to align these sequences
00:07:38.20 at the nucleotide level
00:07:40.12 than it is to align them at the amino acid,
00:07:43.03 where my name can change to a popular car model
00:07:45.11 very quickly,
00:07:46.29 because every mutation
00:07:48.29 has a high likelihood of altering the amino acid
00:07:51.03 being encoded,
00:07:52.21 and this is exactly the signature we see
00:07:54.14 when you have an interface
00:07:56.19 that is precisely at the interface
00:07:58.08 between a host and a virus conflict,
00:07:59.29 and that's because every single one of
00:08:02.05 these amino acid mutations
00:08:04.19 is potentially beneficial
00:08:06.01 and has been acted upon by natural selection
00:08:09.07 to increase their rate of fixation in the population,
00:08:12.15 hence the term diversifying selection.
00:08:15.11 In contrast to purifying selection,
00:08:17.08 natural selection is increasing
00:08:19.10 the amino acid diversity
00:08:21.07 of these protein-coding genes.
00:08:22.27 As a result, what we have, again,
00:08:24.22 is an apparent rate of replacement changes,
00:08:26.24 kA or dN,
00:08:28.13 which is increased
00:08:30.12 over the apparent rate of synonymous changes.
00:08:32.17 Once again, this is not a bias
00:08:34.25 that is introduced by mutation.
00:08:36.02 This is simply a different selective sieve
00:08:38.08 that is acted upon by natural selection.
00:08:41.15 This term diversifying selection
00:08:43.22 is also referred to as positive selection
00:08:45.19 or adaptive evolution.
00:08:47.03 I'll use these terms interchangeably,
00:08:48.29 and they're only different in the context of the tempo
00:08:51.02 with which these changes happen.
00:08:53.14 Now, if you were to take these characteristics
00:08:55.25 of replacement rates and synonymous rates
00:08:57.29 and calculate them for all genes
00:08:59.29 that we can compare between three sets of species,
00:09:02.25 our own species genome,
00:09:04.26 the rhesus macaque,
00:09:06.18 or the chimpanzee genome,
00:09:08.16 what we have is this very nice histogram
00:09:11.02 which really reflects the selective constraints
00:09:13.20 that have acted on all the protein-coding genes
00:09:16.10 within our genome.
00:09:18.01 What you'll see is there's a large number of genes
00:09:20.24 in the left-hand side of this histogram,
00:09:23.01 which means for the bulk of the genes in the human genome,
00:09:25.13 purifying selection,
00:09:27.04 or a dearth of replacement changes,
00:09:28.28 is really what is going on.
00:09:30.19 We are very interested in this sort of
00:09:32.26 small blip of genes right here
00:09:35.04 where you actually have a very small set of genes,
00:09:37.16 which even at the whole-gene level
00:09:39.09 have undergone much faster replacement changes,
00:09:41.10 almost breaking the speed limit of evolution, if you will,
00:09:44.16 to increase because of this diversity.
00:09:46.16 And when you take a really close look at
00:09:48.25 this category of genes,
00:09:50.13 immunity genes are really overrepresented,
00:09:52.06 as you might expect,
00:09:53.19 because these genes have been acted upon
00:09:55.14 repeatedly by natural selection.
00:09:57.21 So, we're going to consider
00:09:59.08 a very specialized case of an arms race
00:10:01.08 in today's seminar,
00:10:03.07 and this arms race ensues when a viral protein
00:10:05.14 begins to antagonize an antiviral protein,
00:10:08.21 and in this example the viral protein antagonism
00:10:11.08 is going to force the antiviral protein
00:10:13.11 to evolve to a state which this viral protein
00:10:16.27 can no longer defeat,
00:10:18.14 which will now force this viral protein
00:10:20.01 to evolve rapidly in order to restore its antagonism.
00:10:22.22 And this, in a microcosm,
00:10:24.27 is one step of this arms race,
00:10:26.29 where both the host protein
00:10:28.26 as well as the viral proteins have evolved
00:10:30.27 in these subsequent arms race interactions.
00:10:33.24 Now, what we're going to consider today
00:10:35.29 is a specialized example of this antagonism,
00:10:38.00 when the viral that is being used to antagonize
00:10:42.02 the host antiviral protein
00:10:43.28 is itself a host protein.
00:10:46.05 So, we are now basically considering
00:10:48.02 how would the host be able to distinguish
00:10:50.11 between an antagonism
00:10:52.07 that is caused by a viral mimic
00:10:54.12 versus its interaction with its own host proteins,
00:10:56.25 and that's the problem we'd like to address today,
00:10:59.04 which is, how do host genomes
00:11:01.12 confront and overcome, if they can,
00:11:04.11 the challenge of pathogen mimicry?
00:11:06.21 In today's seminar,
00:11:08.07 we're going to focus on a very specific example
00:11:10.07 of viral antagonism
00:11:11.18 that is mediated by mimicry,
00:11:13.13 and this example involves the host antiviral protein,
00:11:16.15 protein kinase R (PKR).
00:11:18.08 So, protein kinase R
00:11:20.01 is actually expressed when the organism senses
00:11:22.17 it's under some sort of viral attack
00:11:24.27 by virtue of an interferon detection pathway,
00:11:27.09 but it's actually produced as an inactive monomer,
00:11:29.16 which means it can no longer activate itself
00:11:31.24 as a kinase,
00:11:33.14 which is in the process of putting phosphate moieties
00:11:36.05 onto other proteins.
00:11:37.25 However, if this particular cell
00:11:39.27 happens to be infected by a virus,
00:11:41.23 that is detected by the fact that
00:11:45.01 there will now be double-stranded RNA in the cytoplasm,
00:11:47.05 which should not be case unless the cell
00:11:49.19 was under viral attack,
00:11:51.09 and what PKR will do is
00:11:53.08 it will use the signature of double-stranded RNA
00:11:55.06 to dimerize and activate itself as a kinase
00:11:57.22 whose primary substrate
00:11:59.21 is this protein eIF2α,
00:12:01.24 which stands for elongation initiation factor 2α,
00:12:05.04 which is a very important control step
00:12:07.22 to initiate protein production through the ribosome.
00:12:10.22 However, when PKR will phosphorylate eIF2α,
00:12:13.25 this essentially blocks protein production.
00:12:16.08 So, the cell's response to detecting itself
00:12:19.17 under viral attack is,
00:12:21.07 "I'm going to stop all protein production
00:12:23.05 so that I do not become a virus production factory."
00:12:26.04 This can be a very effective
00:12:27.29 and a very potent block to viral production,
00:12:30.17 and so what viruses have had to come up with
00:12:33.12 is several clever means by which
00:12:35.25 they can actually inhibit the PKR reaction.
00:12:37.21 Some viruses, for instance, inhibit the dimerization of PKR.
00:12:40.27 Some viruses will actually hide away
00:12:43.08 all the double-stranded RNA they produce,
00:12:45.02 whereas some viruses actually
00:12:47.08 will encode a phosphatase that specifically
00:12:49.23 takes out the phosphate residue
00:12:51.28 that is put on by PRK,
00:12:53.15 and perhaps the cleverest model
00:12:55.20 comes from the hepatitis C viruses
00:12:57.25 that actually allow PKR to block protein production,
00:13:00.15 to essentially block all manner of host protein production,
00:13:03.15 but will now nonetheless
00:13:05.20 carry on their own protein production
00:13:07.15 in an eIF2α-independent fashion,
00:13:09.20 really highlighting the clever inventions
00:13:12.00 that are really forced upon
00:13:13.27 by virtue of these Darwinian arms races.
00:13:15.25 In todays' seminar, we're actually going to focus on
00:13:18.03 only one of these antagonists,
00:13:20.02 which is encoded by the poxvirus class proteins,
00:13:23.10 which include smallpox and vaccinia virus,
00:13:26.11 and this is a protein called K3L,
00:13:28.14 which acts as a competitive and non-competitive inhibitor,
00:13:31.08 essentially breaking the interaction
00:13:33.12 between PKR and eIF2α,
00:13:36.12 which basically allows the virus to restore protein production
00:13:40.02 and go on with its life cycle.
00:13:42.05 So, we actually started this by looking at what this arms race,
00:13:45.12 with the potential for multiple antagonists from viruses,
00:13:48.15 has done to PKR evolution.
00:13:50.19 And so, to do this,
00:13:52.15 we actually sequenced the PKR gene
00:13:54.10 from a panel of primates,
00:13:56.03 which includes homonoids,
00:13:57.22 including humans, great apes, as well as gibbons,
00:13:59.28 old world monkeys,
00:14:01.20 which includes things like rhesus macaques,
00:14:03.14 and new world monkeys,
00:14:05.05 which are primates that populate Central and South America.
00:14:07.24 And when we do the sequence,
00:14:09.28 we can actually reconstruct the evolutionally history
00:14:12.25 of essentially every step and every codon
00:14:15.08 across the PKR phylogeny,
00:14:17.04 and so what we see in these numbers here
00:14:19.21 are those dN/dS or kA/kS signatures
00:14:22.24 that I talked about.
00:14:24.19 When when we have very low numbers
00:14:26.22 like this number 0.2, here,
00:14:28.19 that's an indication of not very much happening
00:14:30.19 at the protein evolution level.
00:14:32.11 In contrast, we have some amazing examples
00:14:34.16 like this lineage in old world monkeys,
00:14:36.18 where we actually have 22 replacement changes
00:14:39.17 without a single synonymous change happening.
00:14:42.08 That's a really profound signal
00:14:44.06 of multiple staccato replacement changes
00:14:46.17 occurring in the course of evolution,
00:14:48.14 in a very, very short time frame,
00:14:50.09 really highlighting the very intense
00:14:52.16 and very episodic evolutionary pressures
00:14:55.11 that have acted on PKR
00:14:57.17 over the course of the last 35 million years
00:14:59.22 of primate evolution.
00:15:01.13 If you were now to sort of turn this around
00:15:03.16 and squish it codon by codon,
00:15:05.20 we essentially get a landscape
00:15:07.24 of how PKR has been influenced
00:15:09.28 by positive selection.
00:15:11.11 All of these tick marks that I've shown
00:15:13.07 above the PKR protein
00:15:15.09 are individual codons that have recurrently evolved
00:15:17.15 under positive selection,
00:15:19.06 and you can see that, in the case of PKR,
00:15:21.25 these are really spread throughout the entire protein motif of PKR,
00:15:25.01 including in the N-terminal domain,
00:15:27.19 in this linker domain or the spacer region,
00:15:29.20 as well as in the kinase domain,
00:15:32.00 which actually carries out the very important step
00:15:34.09 of eIF2α phosphorylation.
00:15:37.26 And the reason we think that there's been such
00:15:40.07 dramatic and such widespread positive selection
00:15:42.15 is because multiple viruses
00:15:44.10 actually antagonize completely different domains of PKR
00:15:47.12 in order to mediate their antagonism of PKR.
00:15:50.12 So, what we're gonna focus on today
00:15:52.19 is just one of these antagonists,
00:15:54.05 which is, again, these poxviral antagonist K3L,
00:15:56.27 that actually specifically antagonize
00:15:59.12 the kinase domain of PKR.
00:16:01.25 So, the reason I've been spending so much time
00:16:03.28 discussing K3L with you
00:16:05.27 is because K3L is a special antagonist.
00:16:08.28 It actually is an evolutionary-derived mimic
00:16:12.24 which used to be eIF2α,
00:16:14.29 which means that at some point in poxviral evolution,
00:16:18.08 poxvirus actually stole eIF2α from a mammalian host,
00:16:22.13 and have whittled it away to become this perfect mimic,
00:16:25.29 in order to break PKR's interaction with the eIF2α substrate.
00:16:30.00 Now, what is really remarkable about this interaction
00:16:32.12 is that it not just happened once
00:16:34.25 in mammals,
00:16:36.08 but it's happened on three separate occasions
00:16:38.27 with three completely independent lineages
00:16:40.24 of double-stranded DNA viruses,
00:16:42.20 each of them acquiring a K3L-like mimic
00:16:44.20 from their own version of eIF2α.
00:16:47.16 So, this really highlights the very, very successful
00:16:50.11 strategy of mimicry that is encoded by pathogens,
00:16:54.09 and really, from an evolutionary standpoint,
00:16:56.16 the strategy of mimicry
00:16:58.19 and overcoming mimicry
00:17:00.05 is a debate that's really been going on
00:17:02.10 for a very, very long time,
00:17:04.02 going back all the way to Henry Walter Bates,
00:17:06.04 who really first detected evidence for mimicry
00:17:09.13 in these butterflies in the Amazon,
00:17:11.24 where we have model butterflies
00:17:14.10 that are basically poisonous,
00:17:16.13 and so they're avoided by predators
00:17:18.22 who can use their coloration patterns
00:17:20.21 as an indication to...
00:17:22.17 as a warning signal to stay away from them,
00:17:24.16 and mimic butterflies
00:17:26.10 that actually don't encode a poison at all,
00:17:28.05 but take advantage of this coloration pattern,
00:17:30.11 and mimic the coloration pattern,
00:17:32.08 to take all the advantages of avoidance from predators,
00:17:35.09 without actually having to encode
00:17:37.07 any of the toxins that are required.
00:17:39.19 Now, this is actually quite a really great strategy
00:17:41.27 for the mimic.
00:17:43.06 It's not so good for the model,
00:17:45.03 because as the mimics start increasing in frequency
00:17:47.03 and the predators start eating more and more butterflies
00:17:48.29 that look like this, but are quite tasty,
00:17:51.12 they will lose their avoidance of the predators,
00:17:53.16 which means that the success of the mimic
00:17:56.08 is directly, inversely correlated
00:17:58.22 with the success of the model.
00:18:01.01 And very much the same thing might be going on
00:18:03.00 at a molecular level, we feel,
00:18:04.27 where eIF2α is acting as a model protein,
00:18:07.17 which is being mimicked by this poxviral mimic K3L
00:18:10.27 in order to defeat
00:18:13.22 the PKR-eIF2α immunity response.
00:18:16.29 So, if you were to sort of rephrase
00:18:18.27 the challenge of mimicry,
00:18:20.22 it is that the PKR kinase domain
00:18:22.29 needs to bind and maintain its interaction with eIF2α,
00:18:26.22 while avoiding its interaction with the mimic,
00:18:29.12 which really is evolutionarily being selected
00:18:31.19 to look like eIF2α
00:18:33.11 from the viral perspective,
00:18:34.28 and you can see in this crystal structure
00:18:37.03 that the structures of the PKR interaction domain
00:18:39.27 between K3L and eIF2α
00:18:42.01 are almost completely super-alignable,
00:18:43.23 so how is it that PKR is able to acquire
00:18:46.23 the ability to discriminate between these two?
00:18:49.12 As I've already told you,
00:18:51.08 one of the strategies that PKR is using is very rapid evolution,
00:18:54.26 it's got that at its disposal,
00:18:56.17 and this is just a sliding window plot of dN/dS
00:18:59.12 over the entire protein of PKR,
00:19:01.15 and what you see here is that
00:19:03.14 there is not even a single domain
00:19:05.20 where the dN/dS signature drops below one,
00:19:07.26 which means pretty much every domain of PKR
00:19:10.06 is evolving under positive selection
00:19:12.06 in this comparison between human and rhesus PKR.
00:19:15.08 It's really remarkable how profound the signal is,
00:19:18.14 because when we compare PKR
00:19:20.06 to its closest relative kinase, PERK,
00:19:22.14 which is not involved in antiviral immunity,
00:19:25.04 you can see that the signature is completely profound
00:19:27.23 of purifying selection,
00:19:29.17 and not of positive selection.
00:19:31.15 And this actually gets even more interesting
00:19:33.06 when you look at eIF2α,
00:19:34.22 which is the substrate for PKR,
00:19:36.25 because eIF2α is so important for translation
00:19:41.02 that it has not tolerated any amino acid changes
00:19:43.07 over the course of evolution.
00:19:44.28 You might be actually wondering where the red line went,
00:19:47.12 and actually the red line is exactly on zero,
00:19:50.05 because no amino acid changes have occurred
00:19:52.12 over the course of primate evolution.
00:19:54.05 So, in a way, you can view this
00:19:56.19 as a very high-stakes game of rock-paper-scissors,
00:19:59.28 except eIF2α is always playing rock,
00:20:03.14 and so it would seem that mimic
00:20:05.25 would have a very, very simple game,
00:20:08.04 which is to mimic an unchanging protein
00:20:10.05 and stay there.
00:20:12.06 We wondered whether that was actually the case,
00:20:13.29 because, first of all, we've actually survived poxviruses,
00:20:16.25 and secondly, this suggested that
00:20:19.23 PKR might have some adaptive routes
00:20:21.18 in order to escape mimicry.
00:20:23.06 Furthermore, if it was the case that K3L
00:20:25.05 was simply evolving to an optimal mimic status,
00:20:28.04 we might actually presume
00:20:30.09 that K3L should now be under purifying selection,
00:20:32.14 having optimized for this role in mimicry.
00:20:35.06 Instead, what we actually find
00:20:36.21 when we compare K3L
00:20:39.00 from a panel of poxviruses,
00:20:40.20 is that, very much like PKR
00:20:42.27 shown here on the host side,
00:20:44.18 which is very rapidly evolving,
00:20:46.08 in contrast to eIF2α which is not,
00:20:48.20 K3L happens to be one of the most [quickly]
00:20:52.09 evolving proteins the poxviral genome.
00:20:54.08 So, this is truly an arms race between
00:20:56.19 K3L and PKR.
00:20:58.03 What makes this arms race really interesting
00:21:00.01 is that they're both really evolving
00:21:02.03 to get the attention of eIF2α,
00:21:03.28 which is not changing at all,
00:21:05.29 and so that's what makes the problem of mimicry
00:21:07.29 really interesting from an evolutionary standpoint.
00:21:11.12 So, we wanted to actually have a system
00:21:13.15 in which we could simply assay
00:21:15.20 for the effects of mutations and evolutionary adaptations
00:21:18.17 in a very facile assay,
00:21:20.11 and we actually took advantage of an assay
00:21:22.12 developed first by Tom Dever and Alan Hinnebusch,
00:21:25.03 who recognized that eIF2α
00:21:27.22 is so slow to evolve
00:21:29.15 that if you actually put human PKR in yeast
00:21:32.06 it will actually bind and phosphorylate yeast eIF2α
00:21:34.25 to cause a growth arrest.
00:21:36.26 Now, in this context,
00:21:38.05 if we now also introduce K3L,
00:21:40.11 we have the situation where K3L
00:21:42.18 can give you a readout of whether it's able
00:21:44.20 to defeat PKR or not,
00:21:46.19 based on whether it can rescue the growth inhibition
00:21:49.08 mediated by the PKR expression.
00:21:51.23 So, Nels Elde,
00:21:53.05 who was a postdoc in the lab,
00:21:54.29 actually took this panel of PKR genes
00:21:57.09 from a panel of different primates...
00:21:59.12 homonoids, old world monkeys,
00:22:01.02 and new world monkeys...
00:22:02.20 and he actually just put it into yeast cells,
00:22:04.24 but he put it in a form which could not be turned on.
00:22:07.14 So, when these yeast grow on glucose,
00:22:09.25 because the PKR gene
00:22:11.19 is put on a galactose promoter,
00:22:13.12 it's silenced,
00:22:15.04 and what you can see is that all of these yeast
00:22:17.01 grow perfectly fine.
00:22:18.16 You can see that, even in this serial dilution across,
00:22:20.17 you basically have no growth inhibition.
00:22:22.27 However, as soon as you turn on PKR
00:22:25.17 by putting all of these yeasts onto galactose plates,
00:22:27.27 you can see no yeast growing here,
00:22:30.27 which means all of these PKR alleles
00:22:32.29 have conserved the property of binding
00:22:35.27 and phosphorylating yeast eIF2α,
00:22:37.26 which is remarkable considering the very large degree
00:22:40.20 of evolutionary divergence that we have seen here.
00:22:43.20 Now, I can tell you that this is all because of eIF2α phosphorylation,
00:22:46.17 because in this yeast,
00:22:48.17 if I engineer a mutation in the phosphorylation site
00:22:51.05 all of the growth inhibition goes away,
00:22:53.06 and that's shown in these two panels here.
00:22:55.04 So now, the really interesting question
00:22:57.00 happens when you introduce the viral antagonist.
00:22:59.28 So, what would you predict
00:23:01.24 would happen here if you now introduced
00:23:04.07 the K3L protein from a poxvirus?
00:23:06.13 In this case, we used the vaccinia virus,
00:23:08.28 and what we find is a completely binary response.
00:23:11.27 In some situations, like in the gibbon PKR case,
00:23:15.04 the introduction of the vaccinia K3L
00:23:17.18 completely reverses the growth inhibition that is going on,
00:23:20.23 whereas in the human case,
00:23:23.01 even the presence of K3L,
00:23:25.00 at roughly equal levels of expression,
00:23:27.06 did not overcome the growth inhibition.
00:23:28.26 So, this is exactly like that cartoon example
00:23:31.08 of those two states between hosts and viruses,
00:23:33.27 and what we have in an evolutionary snapshot
00:23:37.01 of both of those states, w
00:23:38.20 here either the host is winning,
00:23:40.18 in which case the growth inhibition goes on,
00:23:42.24 or the virus in winning,
00:23:44.25 in which case the growth inhibition is completely reversed.
00:23:47.10 Now, these are all assays being done in yeast,
00:23:49.17 but we've actually done exactly the same types of assays
00:23:51.29 in vaccinia cells,
00:23:53.27 where we've actually taken either human cells
00:23:56.01 or gibbon cells
00:23:57.16 or orangutan cells
00:23:59.10 and infected them with either a wild type,
00:24:01.10 fully functional vaccinia,
00:24:03.06 or something in which the K3L
00:24:05.26 specifically had been deleted,
00:24:07.21 and what you'll notice is that,
00:24:09.12 in human cells and orangutan cells,
00:24:11.04 it actually doesn't matter
00:24:13.00 whether you've deleted the K3L gene or not,
00:24:14.27 and that's because these species
00:24:17.02 actually have a PKR that's resistant
00:24:19.00 to the K3L antagonism,
00:24:20.25 whereas in the gibbon case,
00:24:22.15 when you delete K3L, you have this 10-fold drop in fitness,
00:24:25.08 which basically is an indication
00:24:27.15 that K3L from vaccinia
00:24:29.21 is acting as a species-specific antagonist
00:24:32.00 of the PKR response.
00:24:34.07 So, we wondered whether
00:24:36.04 we could actually gain better molecular insight
00:24:38.11 into how is it that K3L is able to adopt these multiple states
00:24:41.27 by looking at the co-crystal structure
00:24:44.09 of PKR's kinase domain and the eIF2α substrate,
00:24:47.25 which was first actually established
00:24:50.04 by Arvin Dar and Frank Sicheri's lab,
00:24:52.21 and in this co-crystal structure,
00:24:54.21 one of the most important motifs for this interaction
00:24:58.00 happens to be this α-helix that I've shown here
00:25:01.06 as the g-helix.
00:25:02.23 This is effectively like a bird perch
00:25:04.23 onto which PKR will sit...
00:25:06.21 the bird perch on PKR on PRK
00:25:08.15 onto which eIF2α will sit down.
00:25:10.13 If you take a closer look at the α-helix,
00:25:12.02 shown here,
00:25:13.22 there are three residues in particular
00:25:15.15 that are making direct contacts with the backbone of eIF2α.
00:25:18.29 Now, I'll remind you that eIF2α
00:25:20.18 is not changing at all, in fact,
00:25:22.17 functionally equivalent between human and yeast,
00:25:25.19 so you would predict actually
00:25:28.04 that these three residues would be completely frozen
00:25:30.05 in evolution,
00:25:31.20 by virtue of the fact that they have to interact
00:25:33.25 with something that is completely frozen itself,
00:25:36.05 but instead what we find
00:25:38.05 is that these three residues represent some
00:25:40.12 of the fastest evolving residues
00:25:42.19 in PKR's kinase domain.
00:25:44.10 So, the very...
00:25:46.00 sort of combination lock
00:25:48.02 that is responsible for binding eIF2α
00:25:50.00 is the lock that is very rapidly changing.
00:25:52.05 So, somehow all of these combinations of residues
00:25:55.04 at the αg-helix
00:25:57.13 have preserved the property of binding eIF2α,
00:25:59.25 and yet are basically under very strong evolution.
00:26:02.07 So, we wondered whether this is in fact
00:26:05.08 a signature of the fact that this is an interface
00:26:08.12 that has been constantly challenged by viral mimicry,
00:26:11.14 and so, to test that,
00:26:12.28 we again returned to our yeast assay.
00:26:14.25 We have human PKR
00:26:16.17 that is able to continue growth inhibition
00:26:19.07 even in the presence of K3L,
00:26:21.07 gibbon PKR
00:26:22.27 that is completely reversed by the presence of K3L,
00:26:25.11 and now, in the gibbon backbone,
00:26:27.07 if we add single amino acid changes
00:26:29.27 from human into gibbon,
00:26:31.26 what we find is that we can completely reverse
00:26:34.20 the susceptibility phenotype
00:26:36.11 into the resistant phenotype.
00:26:38.06 So, this really highlights two things.
00:26:40.09 First of all,
00:26:42.10 the interface between PKR and eIF2α
00:26:44.20 is really a hotspot for positive selection,
00:26:47.07 and individual residue changes,
00:26:49.13 these single steps in the arms race
00:26:52.15 between PKR and K3L,
00:26:54.06 result in a complete reversal
00:26:56.10 from susceptibility to resistance.
00:26:58.11 Now, this also actually revealed to us
00:27:00.17 something else that we had missed earlier,
00:27:02.16 which is, even though the orangutan PKR
00:27:05.17 is completely resistant to K3L mimicry,
00:27:07.27 the orangutan g-helix
00:27:10.20 is not resistant to mimicry,
00:27:13.04 which means some other component
00:27:15.27 of the PKR backbone in orangutan
00:27:17.25 is actually necessary for mimicry,
00:27:19.25 immediately suggesting that there was another solution
00:27:22.09 to overcoming mimicry
00:27:24.11 that was evident in orangutan,
00:27:26.05 and we actually mapped that residue again
00:27:28.09 to a single residue in this helix αE,
00:27:30.20 very far away from this helix αG
00:27:33.09 which I've been telling you about today.
00:27:35.09 And so, very much like we saw
00:27:38.16 in the human/gibbon αG case,
00:27:41.09 individual residue changes between gibbon and orangutan
00:27:44.21 have the ability to switch from susceptible to resistant
00:27:48.09 and resistant to susceptible.
00:27:51.15 So, again, really highlighting
00:27:53.12 the very significant power of even individual mutations
00:27:56.04 in individual residues.
00:27:57.27 In the human case,
00:27:59.25 what we also sort of observed was...
00:28:02.09 this particular residue is very interesting,
00:28:04.14 because it's actually toggled
00:28:06.11 between leucine and phenylalanine
00:28:08.12 throughout mammalian evolution,
00:28:10.09 really reflecting the fact that there's probably
00:28:12.05 a high degree of evolutionary constraint
00:28:14.09 acting on this protein,
00:28:15.29 and yet it's toggling so as to keep one step ahead
00:28:18.16 of this mimic interface.
00:28:20.12 The human PKR actually has a very good helix αE residue,
00:28:23.17 as well as a helix αG residue,
00:28:25.28 especially against vaccinia,
00:28:27.25 and we actually have to mutate all three of these residues
00:28:29.27 in order to convert the resistant human PKR
00:28:32.10 into a susceptible version.
00:28:34.20 So, what have we learned from our examples
00:28:37.24 of PKR overcoming the mimicry of K3L?
00:28:40.17 The first really important lesson we learned
00:28:43.06 is that multiple domains of PKR
00:28:45.08 need to be under rapid evolution
00:28:47.09 in order to overcome mimicry.
00:28:48.27 Again, as I pointed out,
00:28:50.18 this is a rock-paper-scissors game,
00:28:52.11 and if only one particular domain
00:28:54.06 was under rapid evolution,
00:28:55.26 K3L would have a much easier task
00:28:57.17 antagonizing and mimicking this interface.
00:28:59.19 The fact that multiple residues
00:29:01.11 in multiple domains
00:29:03.06 are actually rapidly evolving
00:29:04.26 allows these domains to really take turns
00:29:06.28 in antagonizing...
00:29:08.22 overcoming the antagonism of K3L.
00:29:10.14 And, what appears to be the first evolutionary step
00:29:12.26 when PKR encounters this mimicry
00:29:15.10 is actually a negative affinity,
00:29:17.16 where PKR loses affinity,
00:29:19.11 not just to eIF2α,
00:29:21.12 but also to K3L,
00:29:23.11 and then it restores its affinity
00:29:25.08 by interactions in another domain.
00:29:28.08 So, this also implies that there
00:29:30.24 must be extraordinary flexibility for PKR
00:29:32.26 to basically recognize a substrate
00:29:34.23 that really has undergone no changes
00:29:36.24 over the course of evolution.
00:29:38.18 So, just as an example of this flexibility,
00:29:41.03 here again we've the orangutan G-helix
00:29:43.15 in a gibbon backbone,
00:29:45.24 and you can see this is actually susceptible to mimicry,
00:29:49.00 but you can see here, now,
00:29:50.27 because of the growth of this yeast colony,
00:29:52.25 this is telling us that this particular chimeric version of PKR
00:29:56.03 is also not doing a good job of recognizing its substrate,
00:29:59.24 and yet the orangutan backbone
00:30:02.01 has completely restored the binding to eIF2α
00:30:04.23 as well as overcome mimicry,
00:30:06.15 which means something else
00:30:08.15 in the orangutan backbone was sufficient
00:30:10.15 to restore the weakness of this G-helix
00:30:12.28 over the course of these evolutionary arms races.
00:30:15.16 So, this is great,
00:30:17.06 we've learned rules by which PKR
00:30:19.04 might actually overcome mimicry,
00:30:20.24 but this is also sort of a sobering reminder
00:30:22.21 that this overcoming of mimicry
00:30:24.29
00:30:26.24 comes at a cost. So, if you were to look at the αG helix from PKR
00:30:29.05 and three other kinases,
00:30:31.08 whose primary substrate is eIF2α,
00:30:33.11 we'll notice that PKR
00:30:35.18 is the only kinase where we see this dramatic rapid evolution.
00:30:37.25 We don't see if for these three other kinases,
00:30:40.13 which means these kinases
00:30:42.11 have had the evolutionary luxury
00:30:44.20 to optimize to an optimal binding of eIF2α
00:30:48.15 and essentially stay there,
00:30:50.20 preserve their optimal binding
00:30:52.22 by virtue of purifying selection.
00:30:54.15 PKR no longer has that luxury,
00:30:56.18 because as it gets more and more optimal
00:30:58.20 for eIF2α recognition,
00:31:00.23 it gets more and more susceptible
00:31:02.16 for K3L antagonizing it as a mimic.
00:31:05.28 So instead, PKR's evolutionary solution
00:31:08.17 has been to back away from this optimal mimicry
00:31:11.02 in order to gain more of this adaptive landscape
00:31:13.14 that keeps it one step ahead
00:31:15.28 of the virus in terms of these arms races.
00:31:18.02 This a very important sort of consideration
00:31:20.29 because it's not just antiviral genes that face mimicry.
00:31:24.18 This is a slide in which we show that
00:31:28.16 absolutely essential processes in the cell,
00:31:30.24 the cytoskeleton,
00:31:32.14 membrane trafficking,
00:31:34.01 even the cell cycle and apoptosis,
00:31:36.06 all absolutely fundamental housekeeping processes in the cell,
00:31:38.22 are all hijacked by some form of pathogen mimicry.
00:31:42.04 It's worth considering that...
00:31:44.13 what are the evolutionary pressures that have been placed on all of these processes,
00:31:47.09 as they basically tried to survive the mimicry imposed by the pathogen?
00:31:50.20 And, even though they're acquired
00:31:53.22 really great adaptations to overcome this mimicry,
00:31:56.05 some of these alleles might actually be compromised
00:31:59.05 in terms of their housekeeping function
00:32:01.28 - for the function that they were originally intended for.
00:32:03.29 And so, it's not only the fact that the mimic
00:32:06.15 is actually imposing evolutionary adaptation,
00:32:08.28 it might be pushing some of these genes away
00:32:11.20 from their optimal state for cellular function.
00:32:15.00 So, with that I'm going to end this part of the talk.
00:32:17.21 I'd like to really acknowledge Nels Elde,
00:32:20.03 who was a former postdoc in the lab
00:32:22.04 who has his own lab at the University of Utah now,
00:32:24.11 and two very talented technicians,
00:32:25.27 Emily Baker and Michael Eickbush.
00:32:28.03 And this work was done in collaboration
00:32:30.06 with my colleague Adam Geballe,
00:32:32.07 and Stephanie Child in his lab really did all of the viral work
00:32:35.08 that I've discussed.
00:32:36.27 I'd really like to thank our funding sources,
00:32:38.22 and I thank you for your attention.
Viral Evolution
Concepts: Virus-host co-evolution
- Duration: 22:45
- Downloads
- Subtitles
- Transcript
00:00:01.18 Hello. My name is Harmit Malik,
00:00:03.29 and I'm an evolutionary geneticist
00:00:06.07 studying the molecular arms races
00:00:08.05 between primates and viral genomes.
00:00:10.11 I work at the Basic Sciences Division
00:00:12.10 at the Fred Hutchinson Cancer Research Center,
00:00:14.29 and what we hope to under, simultaneously,
00:00:17.15 is not just the evolutionary rules
00:00:19.23 that govern these interactions between primates
00:00:21.28 and viral genomes,
00:00:23.23 which tells us a lot about how we evolved
00:00:25.21 as well as how the viral pathogens
00:00:27.22 that we interact with evolve,
00:00:29.13 but we also would like to use these rules
00:00:31.13 to design better therapeutic strategies
00:00:33.16 to come up with sort of a better
00:00:35.26 antiviral intervention strategy.
00:00:38.10 So, in the second part of my talk today,
00:00:40.04 I'm going to talk about viral evolution
00:00:42.10 and how viruses might actually adopt
00:00:45.14 completely unexpected pathways
00:00:47.17 in order to evolve in these Darwinian arms races
00:00:49.26 between themselves as well as primate genomes.
00:00:54.12 So, a lot of the work on molecular arms races
00:00:56.20 is actually inspired by the fictional character
00:00:59.04 the Red Queen
00:01:00.22 that was introduced to us by Lewis Carroll in his book
00:01:03.06 "Through the Looking Glass",
00:01:04.26 and he pointed out that it takes all the running you can do
00:01:07.03 to keep in the same place,
00:01:08.28 which is what the Red Queen said to Alice.
00:01:11.04 This was actually recognized as
00:01:13.00 a really powerful evolutionary theorem
00:01:14.23 by the evolutionary geneticist Leigh Van Valen,
00:01:17.27 who formalized this into the Red Queen Hypothesis,
00:01:20.27 and he pointed out that
00:01:23.06 when two systems are basically antagonists of each other,
00:01:25.19 where they're both either competing for the same resources
00:01:28.11 or really taking advantage of each other
00:01:31.00 in order to gain an evolutionary dominant strategy,
00:01:33.22 they're going to be basically be always trying to evolve
00:01:36.21 to get the upper hand
00:01:38.15 in this evolutionary arms race,
00:01:40.11 forcing the other component to evolve.
00:01:42.08 And, essentially, they're just always climbing
00:01:44.12 this staircase over evolutionary adaptation,
00:01:47.00 effectively always staying in the same place.
00:01:49.09 So, there's going to be a temporary winner or a loser,
00:01:51.17 which in the next cycle of adaptation
00:01:53.20 gets switched around.
00:01:55.12 And so, this is a very typical situation
00:01:57.17 as it's seen in host-virus conflicts,
00:02:00.04 because this is exactly the type of situation
00:02:02.04 where we'd expect both the viral genome
00:02:04.04 and the host genome,
00:02:05.22 in order to be in conflict with each other,
00:02:07.22 which is why we actually refer to these
00:02:09.28 as the usual suspects.
00:02:11.06 And so, in this slide cartoon here,
00:02:13.06 we have a situation where the host protein
00:02:15.17 is either recognizing a viral protein
00:02:17.17 such that it can actually degrade it
00:02:19.15 and cleanse the organism of this infection,
00:02:22.00 or a viral protein actually acquiring
00:02:24.16 a single amino acid mutation
00:02:26.29 that allows it to evade detection by the immune system,
00:02:29.01 and thereby basically gains an advantage
00:02:31.15 by virtue of no longer being recognized.
00:02:33.23 And so, this is a situation in which
00:02:36.13 either the host or the virus is always losing this arms race,
00:02:39.26 and therefore there's always going to be
00:02:42.01 an evolutionary advantage to be gained by innovation.
00:02:44.13 Now, in a classical Darwinian sense,
00:02:46.14 this arms race can actually by typified
00:02:48.25 in a cartoon example
00:02:50.22 in a population sense.
00:02:52.03 So, here we have again, a very similar situation,
00:02:53.28 an immune surveillance protein,
00:02:55.24 let's say it's an innate immune defense gene,
00:02:58.06 which is actually surveying a population
00:03:00.17 of viruses represented by these coat proteins.
00:03:03.10 Now, very much like Darwinian selection,
00:03:05.19 a random mutation will arise,
00:03:07.24 which might actually affect
00:03:09.22 one of these coat proteins
00:03:11.11 such that it happens to have a mutation
00:03:13.13 that no longer allows it to be recognized
00:03:15.12 with the immune surveillance system.
00:03:17.12 This mutation could be extremely rare,
00:03:19.12 happening in one in a billion viruses.
00:03:21.28 Nonetheless, because all the other viruses
00:03:24.03 are being recognized
00:03:26.15 and cleansed out by the immune system,
00:03:28.11 very quickly this virus is going to take over the population,
00:03:31.01 and now the host is confronted
00:03:33.17 with a virus that is actually a variant,
00:03:35.23 and specifically a variant in an interaction interface,
00:03:39.19 forcing the host genome
00:03:41.22 to now come up with a counter-evolution strategy
00:03:44.09 which involves changes in the host protein.
00:03:46.29 So, most of the positive selection,
00:03:49.20 or most of the evolutionary adaptation,
00:03:52.02 that we've been considering
00:03:54.14 between hosts and viruses
00:03:56.16 has really been focused on these
00:03:58.19 very rapid amino acid replacements
00:04:00.21 in the host-virus interaction interface,
00:04:03.02 but today I'm actually going to tell you about
00:04:05.02 a completely novel strategy that viruses might use,
00:04:08.17 which actually has been hidden from view
00:04:10.26 from a lot of evolutionary biologists,
00:04:12.11 and we could actually capture that
00:04:14.07 by virtue of laboratory experiments
00:04:16.16 that could actually capture all stages
00:04:18.20 of this adaptation process
00:04:20.11 as it happened in a virus.
00:04:22.11 Before I tell you about that, I need to introduce
00:04:24.20 the particular system I'm going to describe,
00:04:26.08 and that's an antiviral gene
00:04:27.28 called protein kinase R, or PKR,
00:04:30.02 which is a very important innate defense system
00:04:32.06 against viruses.
00:04:33.24 So, PKR is actually expressed as an inactive monomer,
00:04:37.29 which means it's no longer active as a kinase.
00:04:40.15 A kinase is a protein that actually
00:04:42.21 puts phosphate moieties onto other proteins.
00:04:45.00 On interferon production,
00:04:47.00 you make PKR but you don't really mount a response.
00:04:49.09 It actually takes an actual viral infection
00:04:51.19 in that cell
00:04:53.23 in order for PKR to dimerize,
00:04:55.29 using the double-stranded RNA,
00:04:57.25 activate itself as a kinase,
00:04:59.21 and now phosphorylate its substrate eIF2α,
00:05:03.09 or elongation initiation factor 2α,
00:05:06.23 whose phosphorylation
00:05:08.23 will basically block protein production
00:05:10.26 through the ribosome.
00:05:12.18 So, this is a very potent block against viruses.
00:05:14.08 They can no longer go through their life cycle
00:05:16.10 if no protein production is allowed to proceed,
00:05:18.24 and this is basically...
00:05:21.03 they have invented all kinds of strategies
00:05:22.27 in order to block this PKR pathway,
00:05:25.11 including preventing its dimerization,
00:05:27.18 hiding away all the double-stranded RNA,
00:05:29.25 actually reversing this phosphorylation step,
00:05:32.04 as well as a completely eIF2α-independent form
00:05:35.14 of translation initiation.
00:05:37.19 We are very focused in the lab
00:05:40.06 on one particular type of antagonist,
00:05:42.06 which is this K3L antagonist
00:05:44.10 encoded by poxviral genomes,
00:05:46.06 which can essentially break the interaction interface
00:05:49.04 between PKR and eIF2α,
00:05:51.12 and by virtue of that essentially block the PKR pathway.
00:05:55.00 Now, in part one of the seminar,
00:05:56.29 I told you how PKR is actually undergoing very rapid evolution
00:06:00.08 in order to gain one step ahead of K3L.
00:06:03.15 What we also see is this arms race
00:06:05.28 is being played out both on the host side
00:06:08.06 as well as on the virus side,
00:06:10.00 so if you look at K3L among different poxvirus genomes,
00:06:12.26 in this case we compared
00:06:14.28 all the vaccinia proteins to all the smallpox proteins,
00:06:17.21 we have this nice histogram
00:06:19.29 of the rates of protein evolution
00:06:21.24 as they happen along the landscape
00:06:24.03 of the genome of poxviruses.
00:06:26.12 So, a very simple way to look at this histogram,
00:06:29.00 or this bar graph,
00:06:30.21 is that genes on the left-hand side
00:06:32.15 are very slow to evolve at the protein level
00:06:34.13 and genes on the right-hand side
00:06:36.17 are very fast-evolving at the protein level,
00:06:38.14 and K3L happens to be one of the fastest-evolving genes,
00:06:41.14 at the protein level, in poxviral genomes,
00:06:44.05 which means this very intense arms race
00:06:46.11 that has played out between PKR and K3L
00:06:48.20 has not only rapidly changed PKR in primate genomes,
00:06:52.19 but has also changed K3L
00:06:54.18 in [viral] genomes.
00:06:57.12 So, we wanted to actually capture the stages of adaptation,
00:06:59.21 and so to do that we actually turned to an
00:07:01.24 experimental evolution strategy,
00:07:03.12 really a very successful strategy
00:07:05.10 in terms of capturing evolutionary states
00:07:07.24 that might be very transient
00:07:09.21 and very difficult to capture in the wild.
00:07:11.25 This is a very important strategy
00:07:13.21 that's been very successfully used, for instance,
00:07:15.18 in bacterial evolution.
00:07:17.15 So, we took the vaccinia virus
00:07:19.14 and we actually made one change in that virus,
00:07:21.29 which is we knocked out this E3L gene,
00:07:24.20 which I've not introduced to you yet so far.
00:07:27.16 E3L is one of those proteins
00:07:29.13 that actually helps hide away the double-stranded RNA
00:07:32.17 to prevent the PKR activation,
00:07:35.12 so the reason we actually E3L
00:07:37.13 was we wanted to put all the selective pressure
00:07:39.21 to overcome the PKR response
00:07:41.28 onto the K3L gene,
00:07:44.03 and we knew, before we started the study,
00:07:47.07 that the vaccinia K3L gene
00:07:49.07 is actually ineffective at defeating the human PKR,
00:07:52.15 which is why, when you delete the E3L protein,
00:07:55.07 we have this dramatic drop in fitness
00:07:58.10 where the wild type [virus],
00:08:00.08 which contains E3L,
00:08:02.00 is almost 1000-fold better at infecting HeLa,
00:08:04.15 or human cells
00:08:06.04 than is this ΔE3L virus,
00:08:08.19 which has been deleted for the E3L gene.
00:08:10.27 So, what we decided to do
00:08:12.22 was simply take this virus
00:08:14.21 and passage it on a plate of HeLa cells,
00:08:17.16 and what happens when these viruses propagate
00:08:20.25 is that you basically make these small plaques,
00:08:23.28 which is where the virus has actually infected
00:08:25.25 and burst through and made more progeny viruses,
00:08:28.11 and we simply take all of these viruses
00:08:30.16 as they emerge from a plate
00:08:32.10 and transfer them to a new plate...
00:08:34.04 except, in the experimental evolution strategy,
00:08:36.20 we always take a historical record of this adaptation
00:08:39.26 by measuring the replication rate
00:08:42.00 at every step of this evolution,
00:08:43.27 as well as saving a fossil record of these viruses
00:08:46.15 at every stage of their adaptation.
00:08:48.18 So, when we now move these to new plates,
00:08:50.27 what we would hope to see is that the virus is getting better,
00:08:53.29 so we're going to see more and more plaques
00:08:55.19 as this virus learns to adapt to HeLa cells.
00:08:58.18 As a very important aside,
00:09:00.09 vaccinia virus
00:09:02.20 being passaged in chicken cells
00:09:04.13 was the basis for the smallpox vaccine,
00:09:06.17 which was responsible for perhaps saving
00:09:09.02 more lives than any other medical intervention
00:09:11.07 that we know of.
00:09:13.00 And so, what we did was simply passage these
00:09:15.18 in HeLa cells for about 10 passages,
00:09:17.17 and in just 10 passages
00:09:19.29 we observed something quite dramatic.
00:09:22.00 So, remember, the wild type fitness is about here,
00:09:26.20 the ΔE3L virus is about here,
00:09:28.20 and what we see is that, although all these viruses
00:09:30.26 started off really poor at infecting HeLa cells,
00:09:33.19 almost all of them, by 10 passages,
00:09:35.26 have really gained most of the fitness that they had lost
00:09:38.27 in terms of their HeLa cell infectivity.
00:09:42.03 So, we actually have multiple ways to test this.
00:09:44.12 This is actually a virus titer assay,
00:09:46.05 in which we see what the progeny virus count looks like,
00:09:49.28 but we've also replaced the E3L gene
00:09:52.06 with a β-galactosidase reporter gene,
00:09:54.09 and we actually measure levels of that reporter gene
00:09:56.13 as another means of actually assaying
00:09:59.00 how successful the virus is,
00:10:00.27 and both of these assays are very, very consistent
00:10:02.26 with each other,
00:10:04.28 suggesting that you started off with a very poor virus
00:10:06.24 and you've actually gained most of the infectivity back
00:10:09.18 in just 10 passages.
00:10:11.09 And so, what kind of rapid evolution
00:10:13.08 might have actually happened,
00:10:14.27 in the course of just 10 passages,
00:10:16.20 for vaccinia to have regained
00:10:18.12 most of the infectivity that it lost?
00:10:20.15 And so, to actually address the genetic basis
00:10:22.28 of how this happened,
00:10:24.16 we decided to actually take the parental strain
00:10:27.12 and sequence it to completion,
00:10:29.11 which means get very high, in-depth sequence coverage
00:10:33.15 to understand, okay,
00:10:35.12 what is the role that perhaps rare mutations are playing
00:10:37.18 in this adaptation?
00:10:39.09 Then we took these three replicates at passage 10
00:10:41.17 and sequenced them
00:10:43.15 such that we could compare.
00:10:45.00 Why are these three replicates
00:10:46.19 so much better than the parental strain
00:10:48.14 in terms of coming up with the solution
00:10:50.25 to HeLa infectivity?
00:10:52.26 So, when we first actually did this...
00:10:55.20 so, we could actually do this with very high coverage
00:10:57.17 because the vaccinia genome is about 200 kB,
00:11:00.12 and with advances in genome sequencing technology
00:11:03.09 we could essentially get about 1000-fold coverage
00:11:06.00 for every nucleotide of the vaccinia genome,
00:11:09.04 which means for any mutation at the level of 1%,
00:11:12.10 we can be very confident
00:11:14.09 that we are not going to miss it,
00:11:15.22 which is really what we wanted
00:11:17.07 to understand the basis for this evolutionary adaptation,
00:11:19.12 but, actually, the first returns
00:11:21.17 were very disappointing.
00:11:23.09 So, although we did see some really nice mutations in K3L,
00:11:26.20 which I'll return to,
00:11:28.23 we actually saw very low mutation
00:11:31.17 across the entire genome,
00:11:32.29 and that's actually consistent with the idea that vaccinia,
00:11:35.06 unlike other RNA viruses like influenza or polio,
00:11:38.03 is a very slowly-evolving virus.
00:11:41.08 So, we wondered, how is it that the virus,
00:11:43.16 which actually didn't acquire a lot of mutations,
00:11:45.24 and very few of the mutations that actually shared...
00:11:48.08 none, in fact, are shared across all three replicates...
00:11:51.10 how did it acquire this dramatic fitness gain
00:11:54.05 despite actually not having been able to
00:11:57.20 explore a lot of the mutation space,
00:11:59.10 for instance, that a rapidly-evolving RNA virus
00:12:01.10 might be able to do?
00:12:03.03 And so, this is the sort of conundrum
00:12:05.03 that really we were stuck at for a little while,
00:12:07.03 until a couple of people in the lab
00:12:10.13 really recognized
00:12:12.12 that we're actually only looking at some of the data
00:12:14.06 by looking at each individual mutation.
00:12:16.07 We have another readout
00:12:17.18 when we do these kinds of genome sequences,
00:12:19.25 which is we can look at how well is one part of the genome
00:12:23.06 represented across the entire sequence read.
00:12:25.25 So, for instance,
00:12:27.19 what we have here is an average genome coverage,
00:12:30.05 normalized to 1,
00:12:31.16 across the entire vaccinia genome.
00:12:34.00 You will see this very interesting blip right here,
00:12:36.13 and this is where we've actually deleted the E3L gene,
00:12:40.02 and so that's exactly what you'd expect
00:12:42.07 if the E3L gene is now missing
00:12:44.08 from what we are comparing to,
00:12:45.27 which is the reference sequence.
00:12:47.13 What really caught our eye, though,
00:12:49.02 was this dramatic blip upwards,
00:12:51.04 and when we took a closer look at these,
00:12:53.08 what these are are independent expansions
00:12:56.03 of the K3L gene
00:12:57.22 in every single replicate,
00:12:59.27 but not in the parental strain.
00:13:02.07 So, you can see the parental strain, shown here in blue,
00:13:05.11 is completely on the genomic average of 1,
00:13:07.12 exactly like its neighboring regions,
00:13:09.06 whereas every single one of the replicates
00:13:11.05 has an average K3L copy number between 3 or 4,
00:13:15.08 which is sort of a really dramatic example
00:13:18.00 of how each of these three replicates
00:13:20.08 has independently converged on the same evolutionary strategy,
00:13:23.14 which is to amplify K3L.
00:13:25.19 We were then wondering whether, basically,
00:13:28.05 we had viruses in here
00:13:30.10 that each have about 3 copies of K3L,
00:13:32.12 and it's a pretty homogenous population,
00:13:34.16 but now that we had the fossil record,
00:13:37.05 we could ask not only what the basis of this expansion was,
00:13:40.06 but when it occurred over the course of evolution.
00:13:43.18 And so, what we discovered when we did this fossil record
00:13:46.08 was we started with a parental virus that had no K3L expansions,
00:13:50.01 and for about 4 passages,
00:13:52.01 really we didn't see very much,
00:13:54.11 but as we went from passage 4 to 10,
00:13:56.20 we have this very heterogeneous virus population
00:14:00.09 with this accordion-like expansion of the K3L gene,
00:14:03.21 where you started with one gene
00:14:05.17 and now you've been ratcheting it upwards
00:14:07.19 with every passage,
00:14:09.16 increasing the average copy number,
00:14:11.13 but the average copy number is actually hiding the fact
00:14:14.01 that there are some viruses in here
00:14:16.04 who have undergone a 10% genome expansion,
00:14:19.15 which is a dramatic expansion for a virus,
00:14:21.28 where real estate is a really important criteria,
00:14:24.25 and they're doing so only focused on the K3L gene,
00:14:28.08 because that is the evolutionary strategy
00:14:30.12 they have come up with
00:14:32.09 to overcome this PKR response.
00:14:34.21 So, another way to actually describe what we see
00:14:37.05 is that we've been able to molecularly map the breakpoints,
00:14:40.11 they flank this K3L gene shown here,
00:14:42.24 and what we basically have is an accordion-like amplification
00:14:45.21 of this original duplication,
00:14:47.18 now to sometimes 15 copies
00:14:49.27 in these heterogeneous viruses.
00:14:51.26 So, this is a very dramatic
00:14:53.22 and very recurrent expansion.
00:14:55.22 I'm only showing you one of the three replicates we did,
00:14:57.21 but the other two replicates look almost exactly identical.
00:15:01.16 So, the fact that this expansion is so recurrent and so dramatic
00:15:04.29 led us to ask, what are the consequences
00:15:07.13 of this expansion?
00:15:08.27 So, we had multiple genes...
00:15:11.00 are they actually making a lot more protein
00:15:12.26 than what you'd expect?
00:15:14.11 And, indeed, to test that,
00:15:15.29 we actually took these passage 10 viruses
00:15:18.00 and transfected them again back...
00:15:20.04 infected them into HeLa cells,
00:15:22.03 and indeed what we see
00:15:24.01 is they are making a lot more K3L
00:15:26.05 than even wild type virus,
00:15:28.23 and if you actually blow up this picture
00:15:30.28 you can see that the parental E3L gene
00:15:33.09 is making very little K3L
00:15:35.02 compared to what is now being made
00:15:36.29 by virtue of this genomic accordion expansion
00:15:39.14 in these replicate (passage) 10 viruses.
00:15:42.13 We can now ask, okay,
00:15:44.00 we now have this K3L expansion,
00:15:46.01 is this the reason
00:15:47.19 why we're seeing this massive increase in fitness?
00:15:49.16 And, to do that,
00:15:51.05 what we did was a strategy in which
00:15:53.01 we can take small interfering RNAs
00:15:55.05 and essentially get rid of most of the K3L RNA
00:15:58.06 that is being produced in these infected cells.
00:16:00.23 And so, when we do that,
00:16:02.20 we can design RNAs and then infect vaccinia,
00:16:05.17 and when we see that what we can see is
00:16:08.02 that these siRNAs are quite effective.
00:16:10.10 So, here's the non-siRNA-inhibited replicate C,
00:16:15.12 at passage 10,
00:16:16.24 and here are a multitude of different siRNAs
00:16:18.28 that basically, to a different degree,
00:16:21.09 knock down the total levels of proteins,
00:16:23.16 and when we compare the fitness
00:16:25.09 of these knockdowns,
00:16:27.25 versus no knockdown or a scrambled siRNA,
00:16:30.02 we can see that when you knock down the K3L protein production
00:16:34.04 you essentially knock down all the gains of fitness
00:16:36.24 that you've gained over the passage 10.
00:16:38.29 So, this K3L accordion-like expansion
00:16:41.22 is both necessary and sufficient,
00:16:45.17 really, to explain this massive increase in fitness
00:16:47.21 that we saw in our laboratory.
00:16:49.29 So, there is of course the trade-off...
00:16:51.28 I mean, these are viruses that actually
00:16:54.09 usually prefer really compact genomes,
00:16:56.15 and the tradeoff is really apparent
00:16:58.10 when we make a comparison of
00:17:01.00 these passage 10 viruses in HeLa cells
00:17:03.01 versus hamster cells.
00:17:04.24 You can see, in HeLa cells,
00:17:06.15 each of the replicate viruses
00:17:08.08 is doing a lot better
00:17:10.04 than the parental virus,
00:17:11.19 whereas in hamster cells,
00:17:13.10 these viruses are actually doing worse
00:17:15.08 than the parental virus.
00:17:16.18 So, what's going on?
00:17:18.12 It turns out that vaccinia K3L,
00:17:20.05 at the starting point,
00:17:22.00 is ineffective to defeat human PKR,
00:17:24.01 and it needs this massive gene expansion
00:17:26.09 in order to overcome, biochemically,
00:17:28.22 the inhibition encoded by the PKR protein,
00:17:31.17 whereas even a single-copy vaccinia
00:17:33.22 is able to overcome
00:17:35.29 the PKR inhibition encoded by hamsters.
00:17:39.03 And so, what we have now begun to see is,
00:17:41.16 if you take this accordion-expanded virus
00:17:44.06 and now infect BHK, or hamster cells,
00:17:47.12 the accordion has now begun to collapse,
00:17:49.11 by virtue of the fact that
00:17:52.03 the fitter virus is actually the smaller virus.
00:17:54.00 And so, this is an example
00:17:55.26 where we've got this transient expansion in HeLa cells,
00:17:58.01 which is now going the opposite way
00:18:00.01 in hamster cells.
00:18:01.23 So, I'll return to those mutations
00:18:03.18 that we actually first detected,
00:18:05.06 which were so disappointing because they were not recurrent,
00:18:08.03 but one of those mutations was especially interesting
00:18:10.17 to us because it occurred in the K3L gene.
00:18:13.02 It's present at about 3% frequency in replicate A
00:18:16.00 and at 12% frequency in replicate C.
00:18:19.18 This is a mutation in the 47th amino acid,
00:18:22.07 changing a histidine to an arginine.
00:18:24.25 The reason this is really interesting
00:18:26.25 is because a completely independent assay
00:18:29.05 many, many years earlier,
00:18:31.05 from Tom Dever's group, had done a yeast selection experiment
00:18:34.16 in which they wanted to ask,
00:18:36.18 can we do a mutational experiment
00:18:39.10 asking, what mutation in K3L can actually overcome
00:18:42.04 the inhibition encoded by PKR
00:18:44.14 and allow this yeast growth to recover?
00:18:47.10 If you want to learn more about this yeast growth assay,
00:18:49.21 I suggest you watch part 1 of the seminar.
00:18:52.14 What is really interesting is they come up
00:18:54.16 with one mutation, H47R.
00:18:57.05 We have done a completely independent experiment,
00:18:59.22 in vaccinia infections,
00:19:01.25 and vaccinia is basically also telling us
00:19:03.28 that this is the evolutionary solution
00:19:06.05 that vaccinia has come up with
00:19:08.04 in completely different assays,
00:19:10.02 both in yeast and human.
00:19:11.27 So, what that means is, now,
00:19:13.12 you started off with a K3L
00:19:15.06 that was not able to defeat human PKR,
00:19:17.03 and now you've acquired a single amino acid mutation
00:19:19.20 that in a Darwinian sense
00:19:21.26 is able to defeat human PKR.
00:19:23.26 But, because we actually now have
00:19:26.05 two forms of adaptation,
00:19:27.26 this gene accordion model that we've discovered,
00:19:30.04 and this classical Darwinian adaptation model,
00:19:32.06 we could now ask, going back to the fossil record,
00:19:34.23 which occurred first,
00:19:36.10 and did one depend on the other?
00:19:38.08 And, when we basically do that,
00:19:40.08 by looking at when one type of mutation
00:19:42.18 occurred relative to the other,
00:19:44.14 what we find is that the expansion
00:19:47.07 actually already happened by passage 4,
00:19:50.00 whereas this mutation actually
00:19:52.08 only began to occur around passage 5 and 6.
00:19:55.01 Moreover, many of these H47R mutations
00:19:57.28 actually occur in these already expanded accordions,
00:20:01.18 which strongly suggests that after the accordion expansion
00:20:05.11 you actually increase the mutational probability
00:20:08.25 of acquiring an H47R mutation,
00:20:11.06 which now, by virtue of even a single copy,
00:20:14.06 is able to overcome the PKR response.
00:20:18.05 So, that actually leads to a very interesting suggestion
00:20:20.22 in terms of how these Red Queen conflicts
00:20:23.02 might actually play out in evolution.
00:20:25.13 We think of the K3L-PKR interaction
00:20:28.24 as a classical Darwinian arms race,
00:20:31.05 so starting off with a step
00:20:34.05 in which the vaccinia K3L is not able
00:20:36.02 to defeat the human PKR,
00:20:38.02 we basically acquired sort of a transient amplification
00:20:41.09 of the K3L gene,
00:20:43.15 which then allowed for the selection
00:20:45.19 of the H47R mutation,
00:20:47.15 which was able to defeat human PKR.
00:20:50.00 Now, what's really interesting
00:20:51.25 is now we have a single-copy gene
00:20:54.11 that is able to defeat human PKR,
00:20:56.08 and we are very interested in asking,
00:20:58.19 now that you've acquired the right mutation,
00:21:00.12 will the accordion collapse to mitigate
00:21:02.10 all the fitness costs of this gene expansion?
00:21:04.21 So, the reason I put this cartoon up
00:21:06.27 is because this cartoon should remind you
00:21:09.02 a lot about this cartoon of the classical arms race,
00:21:11.09 the way we think about in terms of
00:21:14.07 virus antagonizing humans,
00:21:16.00 but we might actually be missing this very, very important
00:21:18.10 transient step which involves gene amplification,
00:21:21.18 especially in viruses and pathogens
00:21:23.18 that actually don't have the high mutation rates
00:21:26.10 necessary to sample the adaptive landscape.
00:21:29.16 And so, very much like
00:21:32.05 the ability for influenza to undergo chromosome reassortment
00:21:36.04 in order to infect new hosts,
00:21:38.10 we think that gene amplification
00:21:40.09 is one of the critical strategies
00:21:42.06 that large double-stranded DNA viruses
00:21:44.06 actually might be using in order to stay...
00:21:47.05 keep pace with this sort of rapid evolution
00:21:49.12 of the host genes that they're actually antagonizing.
00:21:52.22 So, with that,
00:21:54.12 I'm going to acknowledge the people who did the work.
00:21:56.05 All of this work was actually done by a former postdoc in the lab,
00:21:58.20 Nels Elde, who now heads his own lab at the University of Utah,
00:22:02.22 in collaboration with Emily Baker and Michael Eickbush.
00:22:06.09 We do all of our poxviral work
00:22:08.14 in collaboration with my senior colleague Adam Geballe,
00:22:10.20 and I'd especially like to acknowledge
00:22:12.13 Stephanie Child, from his lab,
00:22:14.20 who really did all of the poxviral infection experiments
00:22:16.20 in collaboration with Nels.
00:22:18.15 I'd like to thank Tom Dever,
00:22:20.08 Welkin Johnson,
00:22:21.20 and Michael Emerman,
00:22:23.10 for a lot of reagents and help,
00:22:24.24 and I'd especially like to thank Jay Shendure
00:22:26.24 and Jacob Kitzman, from his lab,
00:22:28.20 for helping us with the analysis of these poxviral sequences.
00:22:31.25 And thank you, I hope you had a good time listening to this.
Related Resources
- Paul E. Turner iBioSeminar: Virus Ecology and Evolution: from virus adaptation to phage therapy
- Harmit Malik iBioSeminar: Molecular arms races between primate and viral genomes
Speaker Bio
Harmit Malik

Harmit Malik received his undergraduate degree in chemical engineering from the Indian Institute of Technology, Bombay and his PhD from the University of Rochester. He moved to the Fred Hutchinson Cancer Research Center in Seattle for post-doctoral work and decided to stay, starting his own lab in the Division of Basic Sciences in 2003. Malik… Continue Reading
Paul Turner
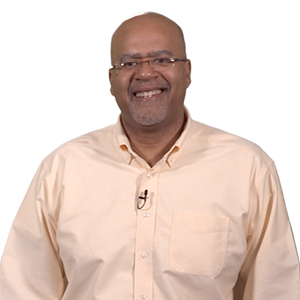
Elihu Professor of Ecology and Evolutionary Biology at Yale University; Professor of Microbiology at Yale School of Medicine
Yale University Continue Reading
Leave a Reply